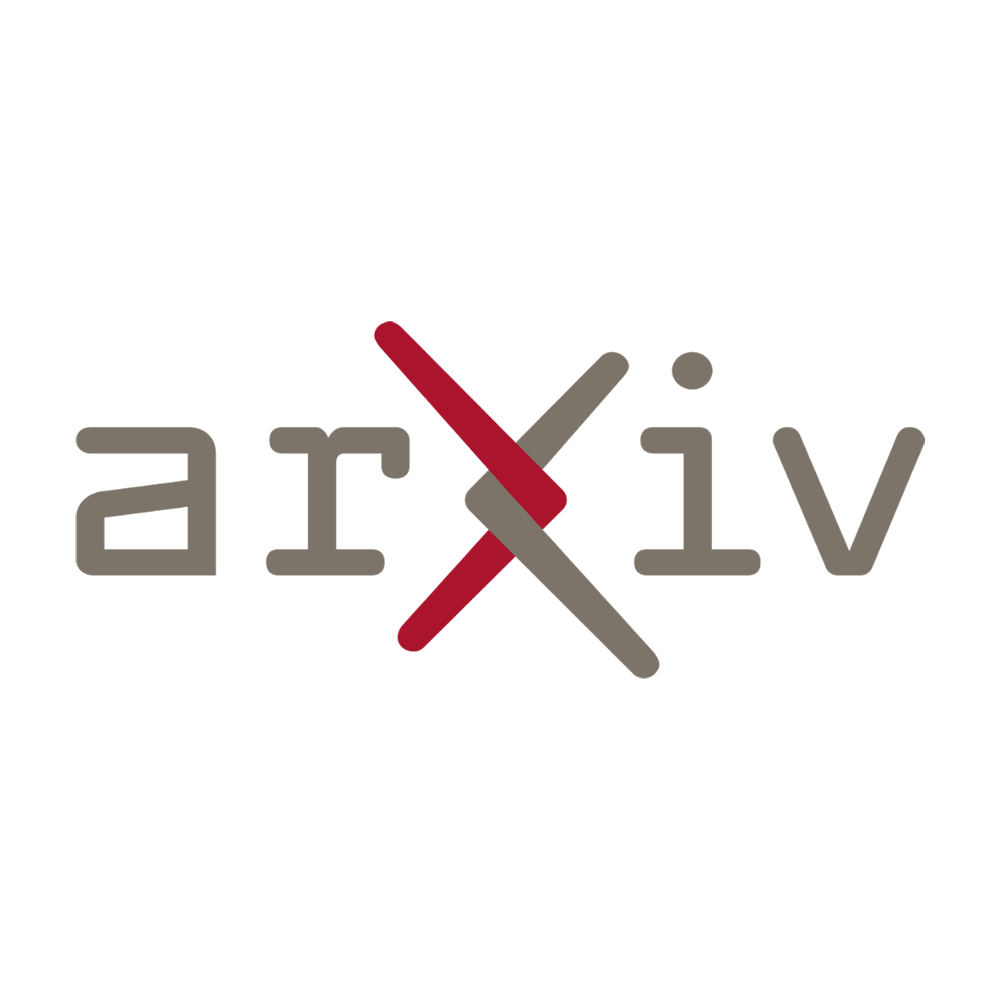
In the realm of action recognition, multimodal-based methods utilizing pose and RGB modality have seen remarkable success. These techniques have allowed for accurate identification and understanding of various actions. However, there are certain limitations that impede the effectiveness of these methods. Skeleton sequences, while providing valuable information about body movements, lack the ability to depict appearance details. On the other hand, RGB images often contain irrelevant noise, making it challenging to extract meaningful action features. To address these shortcomings, researchers are exploring innovative approaches that combine the strengths of both modalities while mitigating their weaknesses. By doing so, they aim to enhance the accuracy and robustness of action recognition systems, paving the way for more advanced applications in fields such as surveillance, human-computer interaction, and sports analysis.
Multimodal Action Recognition: Exploring New Solutions
In recent years, multimodal-based action recognition methods have made significant strides in accurately identifying and categorizing human movements. This has been achieved through leveraging pose and RGB modalities, enabling systems to analyze skeletal data and RGB images simultaneously. While these approaches have seen substantial success, it is essential to acknowledge the limitations of these techniques. Specifically, skeletons sequences lack appearance depiction, and RGB images suffer from irrelevant noise.
The Limitations of Skeletons Sequences
Skeletons sequences provide valuable information regarding human poses and joint movements. Analyzing these skeletal data can capture the underlying actions accurately. However, skeletons alone fail to represent important appearance features such as clothing texture, body shape, and facial expressions. The absence of visual cues limits the expressiveness of the recognized actions and hinders the system’s ability to accurately classify movements that heavily rely on appearance information.
The Challenge of Irrelevant Noise in RGB Images
RGB images offer rich visual representation that significantly helps in recognizing actions. However, RGB images also present challenges due to the presence of irrelevant noise. This noise can arise from factors such as varying lighting conditions, occlusions, and background clutter. These distractions can mislead the recognition system and lead to inaccurate action classification. Addressing the noise issue is crucial to improve the overall performance and reliability of multimodal action recognition systems.
Introducing Innovative Solutions
Recognizing the limitations of current multimodal action recognition methods, we propose innovative solutions that aim to enhance accuracy and address the aforementioned challenges.
1. Combining Texture-based Features with Skeletons
To overcome the lack of appearance depiction in skeleton sequences, we propose integrating texture-based features extracted from RGB images into the skeletal data. By merging appearance information with pose analysis, the system can capture more comprehensive action representations. This combined approach would enable the recognition system to fine-tune its classification based on both skeletal and appearance cues, resulting in improved accuracy and reliability.
2. Noise Reduction Techniques for RGB Images
To mitigate the impact of irrelevant noise in RGB images, we suggest integrating noise reduction techniques into the action recognition pipeline. These techniques might include sophisticated denoising algorithms that remove distractions caused by changing lighting conditions, occlusions, and background clutter. By pre-processing the RGB images before analysis, the recognition system can focus on relevant action features accurately, leading to more accurate action classification results.
The Future of Multimodal Action Recognition
By addressing the limitations of current multimodal action recognition methods, such as the lack of appearance depiction in skeleton sequences and irrelevant noise in RGB images, we can unlock the potential for even more accurate and reliable systems. The proposed solutions of combining texture-based features with skeletons and applying noise reduction techniques to RGB images offer innovative ways to enhance action recognition performance.
“The integration of appearance cues and noise reduction techniques can revolutionize multimodal action recognition, opening new possibilities for diverse applications, including surveillance systems, virtual reality, and human-robot interaction.”
As researchers continue to explore these avenues, we anticipate groundbreaking advancements that will enable multimodal action recognition systems to accurately perceive and understand human movements with greater efficacy and reliability than ever before.
Multimodal-based action recognition methods have undoubtedly made significant strides in recent years, particularly through the utilization of pose and RGB modalities. These approaches have proven to be quite successful in capturing the intricacies of human actions. However, it is important to acknowledge that each modality has its limitations.
One of the primary challenges with skeleton sequences is the lack of appearance depiction. While skeletons provide valuable information about the spatial configuration of body parts and their movements, they fail to capture the fine details of human appearance. This absence of appearance information can sometimes hinder the recognition of certain actions that heavily rely on visual cues, such as handling objects or subtle facial expressions.
On the other hand, RGB images do offer a rich representation of appearance but often suffer from irrelevant noise. This noise can arise from various sources, including cluttered backgrounds, changes in lighting conditions, occlusions, and even variations in clothing or camera viewpoints. Such noise can introduce ambiguity and make it difficult for action recognition algorithms to discern relevant visual features from irrelevant ones.
To address these challenges and enhance multimodal action recognition further, researchers are exploring various avenues. One promising direction is the integration of additional modalities, such as depth or optical flow. Depth information can provide valuable cues about the 3D structure of actions, complementing the spatial information offered by skeletons. Optical flow, on the other hand, captures the motion patterns within a video sequence and can help distinguish between different actions with similar appearances.
Another avenue for improvement lies in leveraging advanced deep learning techniques. Convolutional neural networks (CNNs) have revolutionized image-based action recognition by automatically learning discriminative features from raw pixels. Similarly, recurrent neural networks (RNNs) have been successful in modeling temporal dependencies in sequential data like skeleton sequences. By combining these approaches and designing novel architectures that exploit both appearance and motion information, researchers can potentially mitigate the limitations associated with individual modalities.
Furthermore, attention mechanisms and graph-based models have shown promise in capturing relevant information from multimodal data. Attention mechanisms enable the model to focus on the most informative regions or frames within an input sequence, effectively reducing the impact of noise or irrelevant information. Graph-based models, on the other hand, can capture the complex relationships between body parts or objects involved in an action, allowing for a more holistic understanding of the action dynamics.
In the future, we can expect multimodal action recognition methods to continue evolving and improving. The fusion of multiple modalities, the development of more robust deep learning architectures, and the incorporation of attention mechanisms and graph-based models are all promising avenues for enhancing performance. Additionally, the emergence of large-scale annotated datasets and the availability of powerful hardware will further facilitate advancements in this field. Ultimately, these advancements will lead to more accurate and robust action recognition systems, enabling applications in areas like surveillance, human-computer interaction, and video understanding.
Read the original article