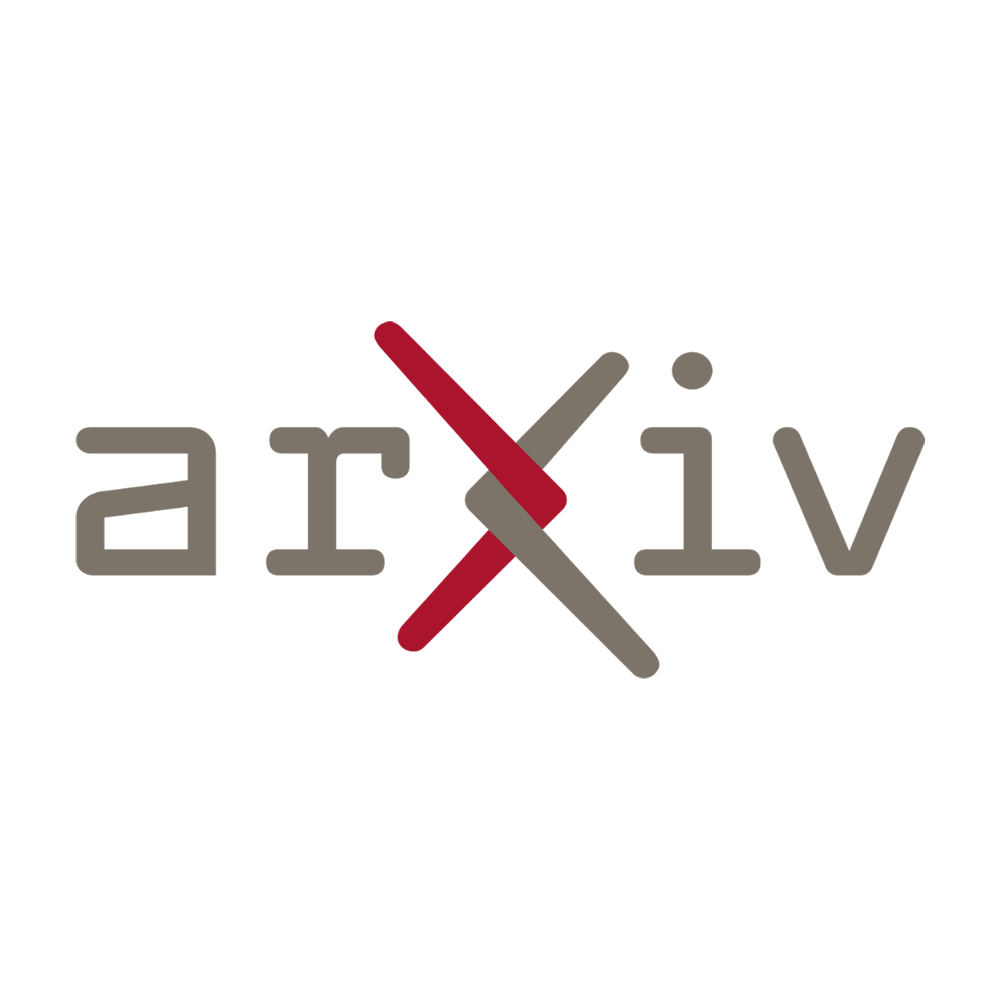
In the field of medical image segmentation, Domain Generalization (DG) has emerged as a crucial technique to address the challenge of domain shifts between different domains. The goal of DG is to achieve high performance on unseen target domains by reducing the disparities between them. This article explores the significance and widespread adoption of DG in medical image segmentation, highlighting its potential to enhance the accuracy and effectiveness of this critical task.
Domain Generalization (DG): A New Approach to Medical Image Segmentation
Medical image segmentation plays a crucial role in the field of healthcare, enabling accurate diagnosis, treatment planning, and disease monitoring. However, the performance of segmentation models often degrades when applied to unseen target domains, due to domain shifts. Addressing this challenge, researchers have embraced the concept of Domain Generalization (DG) to minimize these shifts and improve performance on previously unseen domains.
Understanding Domain Generalization
Domain Generalization focuses on reducing domain shifts by training segmentation models on multiple source domains with different characteristics. Traditional approaches often rely on domain adaptation techniques, which aim to align the source and target domains. However, DG goes a step further by emphasizing generalization across multiple domains, enabling robust performance on previously unseen data.
The key idea behind DG is to identify and utilize common features that are consistent across diverse domains. By effectively leveraging these shared characteristics, segmentation models can learn to generalize well and perform reliably on new domains, even if they differ significantly from the source domains used for training.
Innovative Solutions for DG in Medical Image Segmentation
While DG has shown promising results in various computer vision tasks, including image classification and object detection, its application to medical image segmentation presents specific challenges. Yet, innovative solutions are emerging that push the boundaries of DG in this critical domain.
1. Incorporating Structural Constraints
In medical image segmentation, capturing spatial and anatomical constraints is essential for accurate results. Researchers are exploring ways to incorporate these constraints into the DG framework. By considering the underlying structure of medical images, models can better differentiate between relevant anatomical regions and background noise, even in unseen domains.
2. Generative Models for Synthetic Data Augmentation
Data scarcity is a common issue in medical imaging, making it difficult to obtain large-scale labeled datasets across diverse domains. One innovative solution is to generate synthetic data using generative models like Generative Adversarial Networks (GANs). By training these models on existing data and then augmenting the training set with synthetic samples, DG models can learn from a more diverse range of domains, enhancing their generalization capability.
3. Lifelong Learning and Continual Adaptation
Medical imaging is a dynamic field where new domains and variations constantly emerge. To accommodate these changes, lifelong learning and continual adaptation strategies are being explored. Instead of training models on fixed datasets, these approaches allow models to adapt and learn continuously over time, incorporating new domain knowledge and adapting to evolving patient populations.
The Future of DG in Medical Image Segmentation
The concept of Domain Generalization has opened up exciting possibilities in medical image segmentation. By addressing domain shifts and enabling robust performance across unseen domains, DG holds great promise for enhancing clinical decision-making and improving patient outcomes. As researchers continue to explore innovative solutions and push the boundaries of DG, we can expect significant advancements in this critical field.
Disclaimer: This article is for informational purposes only and does not constitute medical advice. Consult a qualified healthcare professional for personalized diagnosis and treatment.
Domain generalization (DG) is a critical research area in the field of medical image segmentation. The main objective of DG is to address the issue of domain shifts between different domains and ensure that the performance achieved on an unseen target domain remains promising.
In medical imaging, domain shifts are quite common due to variations in imaging protocols, devices, and patient populations across different hospitals or healthcare institutions. These variations can significantly affect the performance of segmentation models when applied to unseen data. Therefore, developing robust DG techniques becomes crucial to ensure the generalizability and reliability of medical image segmentation algorithms.
DG methods typically involve training models on multiple source domains with diverse characteristics and then fine-tuning them on a target domain. The idea is to train the model to learn domain-invariant features that are relevant across different domains. This helps in mitigating the negative effects of domain shifts and enhances the performance of the model on unseen data.
One of the challenges in DG for medical image segmentation is the availability of labeled data from multiple domains. Collecting annotated data from various sources can be time-consuming, expensive, and sometimes impractical. To address this issue, researchers have explored various techniques such as unsupervised domain adaptation and self-supervised learning, which aim to leverage unlabeled data to bridge the domain gap.
Additionally, recent advancements in deep learning architectures have also contributed to improving DG performance in medical image segmentation. Techniques like domain adversarial neural networks, which introduce a domain classifier to encourage the model to learn domain-invariant features, have shown promising results. Moreover, generative adversarial networks (GANs) have been used to generate synthetic data that simulates the target domain, thereby augmenting the available training data and reducing the domain gap.
Looking ahead, there are several avenues for further exploration in the field of DG for medical image segmentation. One potential direction is the integration of transfer learning techniques, where pre-trained models from related tasks or domains can be leveraged to improve the generalization performance. Additionally, incorporating advanced data augmentation techniques, such as domain-specific augmentation, can help in generating more diverse training samples and further reduce the domain gap.
Furthermore, the development of benchmark datasets that encompass a wide range of imaging domains and pathologies would facilitate fair comparisons between different DG methods. This would enable researchers to identify the strengths and weaknesses of existing approaches and encourage the development of novel techniques.
In conclusion, domain generalization plays a crucial role in addressing the domain shift problem in medical image segmentation. The continuous exploration of advanced DG techniques, integration of transfer learning, and the availability of comprehensive benchmark datasets will pave the way for more robust and reliable segmentation models that can generalize well across diverse medical imaging domains.
Read the original article