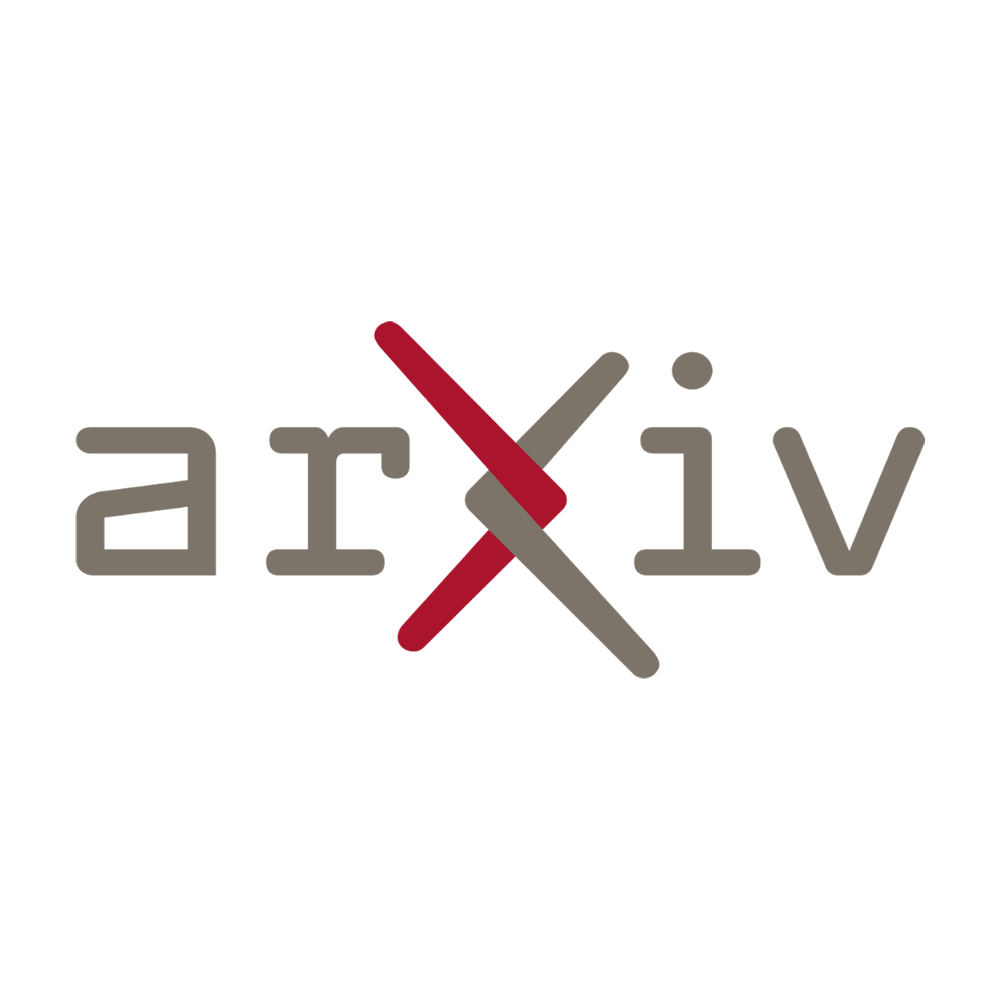
Log anomaly detection is an essential aspect of artificial intelligence for IT operations (AIOps) and plays a crucial role in identifying and addressing irregularities within log data across various domains. This article explores the significance of log anomaly detection and highlights the challenges associated with retraining the entire network to detect unknown anomalies. By delving into the complexities of this field, readers will gain a comprehensive understanding of the importance of log anomaly detection in AIOps and the advancements being made to enhance its effectiveness.
Log anomaly detection plays a pivotal role in the realm of artificial intelligence for IT operations (AIOps). It involves analyzing log data across diverse domains, necessitating the retraining of the entire network to detect unknown anomalies accurately. However, this conventional approach can be resource-intensive and time-consuming, impeding the agility and effectiveness of log anomaly detection systems.
Rethinking Log Anomaly Detection
To innovate log anomaly detection, we must shift our perspective and consider novel approaches that challenge the traditional methodologies. By adopting alternative techniques and leveraging emerging technologies, we can overcome the limitations of the existing systems and forge new paths to more efficient and effective log anomaly detection.
1. Domain-Specific Anomaly Detection Networks
Instead of training a single network for log anomaly detection across various domains, a domain-specific approach can be employed. By designing separate anomaly detection networks tailored to specific domains, we can optimize performance and focus on detecting anomalies that are unique to each domain.
For example, by training a network specifically for web server logs, it can learn patterns and deviations specific to web server behavior. Similarly, a network focused on database logs can better identify aberrations relevant to database operations. This domain-specificity enables greater accuracy and quicker adaptation to new anomalies within a particular domain.
2. Transfer Learning from Related Domains
Transfer learning, a technique commonly used in machine learning, can be harnessed to enhance the agility of log anomaly detection networks. Rather than retraining an entire network from scratch for every new domain, we can leverage knowledge gained from related domains to bootstrap the training process.
For instance, if we already have a well-trained network for web server logs, we can utilize its foundational knowledge when training a new network for application server logs. This accelerates the learning process, reduces the training time required, and improves the overall performance of the log anomaly detection system.
3. Integration of Unsupervised Learning
Unsupervised learning techniques can augment the capabilities of log anomaly detection systems by enabling them to identify anomalies without relying on labeled data. This approach reduces the dependency on annotated log datasets, which can be scarce and time-consuming to create.
An unsupervised learning algorithm can identify anomalies by contrasting patterns in log data to establish what is considered “normal behavior.” Deviations from this norm can be flagged as potential anomalies, providing a more efficient and adaptable log anomaly detection mechanism.
In Conclusion
Rethinking log anomaly detection opens up new possibilities for innovation in AIOps. By employing domain-specific anomaly detection networks, leveraging transfer learning, and integrating unsupervised learning techniques, we can enhance the agility, accuracy, and efficiency of log anomaly detection systems.
Remember: The field of AIOps thrives on ingenuity and the exploration of new approaches. As we challenge established methodologies, we pave the way for transformative advancements in log anomaly detection.
log anomalies can be a challenging task. Log anomaly detection plays a crucial role in AIOps by helping organizations proactively identify and address potential issues within their IT infrastructure. By analyzing log data from various domains, such as system logs, application logs, network logs, and security logs, AI algorithms can identify patterns and anomalies that may indicate abnormal behavior or potential problems.
One of the main challenges in log anomaly detection is the ability to handle log data from diverse sources and domains. Each domain has its own unique log format and structure, making it difficult to generalize anomaly detection algorithms across different types of logs. For example, system logs may contain information about CPU usage, memory utilization, and disk I/O, while application logs may include details about user interactions, transactions, and errors.
To address this challenge, AI models need to be trained on a wide variety of log data from different domains. However, retraining the entire network for unknown log anomalies can be resource-intensive and time-consuming. Therefore, it is important to develop efficient techniques that can adapt and learn from new log data without requiring a complete retraining of the network.
One approach to tackle this issue is transfer learning, which allows the model to leverage knowledge learned from one domain to improve performance in another domain. By pretraining the model on a large dataset of diverse logs and then fine-tuning it on specific domain data, the model can effectively detect anomalies in previously unseen log data.
Another important aspect of log anomaly detection is the ability to distinguish between normal variations and true anomalies. Not all deviations from expected behavior are necessarily indicative of a problem; some may be due to seasonal patterns or expected changes in workload. Therefore, it is crucial for AI models to incorporate domain knowledge and contextual information to accurately differentiate between normal variations and anomalies that require attention.
Looking ahead, advancements in deep learning techniques, such as graph neural networks and attention mechanisms, hold promise for improving log anomaly detection. These techniques can capture complex relationships and dependencies between log events, allowing for better anomaly detection and root cause analysis.
Furthermore, the integration of log anomaly detection with other AIOps components, such as performance monitoring, event correlation, and incident management, can enable a more holistic approach to IT operations. By combining insights from various data sources, organizations can gain a comprehensive understanding of their IT environment and proactively address potential issues before they impact business operations.
In conclusion, log anomaly detection is a critical component of AIOps that helps organizations identify and address potential issues within their IT infrastructure. Overcoming the challenges associated with log data from variant domains requires innovative techniques like transfer learning and incorporating domain knowledge. Advancements in deep learning and the integration of log anomaly detection with other AIOps components will continue to enhance the effectiveness of this field, enabling organizations to achieve more proactive and efficient IT operations management.
Read the original article