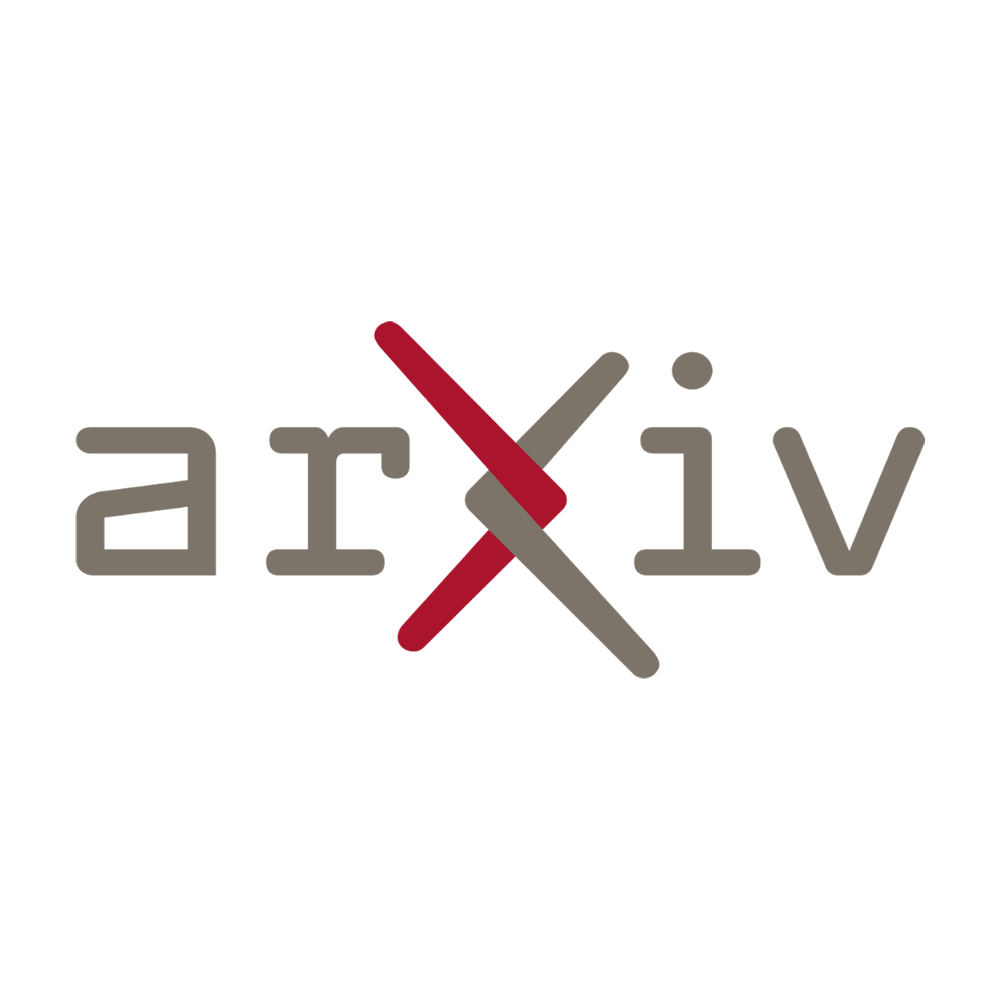
Skin cancer is a pressing issue that affects people worldwide, demanding prompt and precise diagnosis to enhance patient outcomes. In light of this, a groundbreaking study has emerged, introducing an innovative approach to classifying skin cancer. By delving into the core themes of this article, we will explore how this novel methodology could revolutionize the detection and treatment of skin cancer, potentially saving countless lives.
Skin cancer is a global health concern, necessitating early and accurate diagnosis for improved patient outcomes. This study introduces a groundbreaking approach to skin cancer classification, offering innovative solutions and ideas.
The Importance of Early Diagnosis
Early diagnosis is crucial for effective treatment of skin cancer. Detecting skin cancer in its initial stages allows for less invasive treatment options and higher survival rates. However, diagnosing skin cancer accurately can be challenging, often requiring invasive biopsies and expert analysis.
A New Paradigm in Skin Cancer Classification
This study presents a novel approach to skin cancer classification. Instead of solely relying on traditional visual inspection, the researchers developed a technological solution that utilizes artificial intelligence (AI) algorithms and machine learning techniques.
By training AI models with a vast dataset of skin cancer images, the system is able to recognize patterns and characteristics that may not be apparent to the human eye. This transformative technology enables faster and more accurate identification of cancerous lesions.
Advantages of AI-Driven Classification
- Improved Accuracy: The AI-driven classification system surpasses human accuracy in identifying skin cancer. This reduces the risk of misdiagnosis and provides greater peace of mind for both patients and healthcare professionals.
- Efficiency and Speed: The automated classification process significantly reduces the time required for diagnosis. Patients can receive prompt treatment, leading to better outcomes.
- Accessibility: This innovative solution provides access to accurate skin cancer diagnosis in regions with limited healthcare resources. Remote areas or communities with limited medical facilities can benefit from this technology, bridging the gap in healthcare disparities.
Ethical Considerations
As with any technological advancement, there are ethical considerations that accompany AI-driven skin cancer classification.
- Data Privacy: Protecting patient privacy and ensuring the secure handling of medical data is crucial. Robust safeguards must be in place to prevent unauthorized access and use of sensitive information.
- Supplementing, Not Replacing: AI technologies should not replace the expertise of healthcare professionals but rather support and enhance their diagnosis capabilities. The human touch and patient interaction should remain at the forefront of skin cancer management.
- Equitable Access: Introduction of AI-driven classification must ensure equal access for all patients, regardless of socio-economic status or geographic location. Efforts should be made to prevent exacerbating existing healthcare disparities.
A Collaborative Future
“The fusion of technology and healthcare holds immense potential in improving patient outcomes. By embracing AI-driven classification for skin cancer, we can revolutionize diagnostics and make accurate early detection more accessible to all.”
The groundbreaking approach to skin cancer classification presented in this study represents the future of efficient and accurate diagnostics. By harnessing the power of AI, we can provide better patient care, increase survival rates, and reduce the burden on healthcare systems worldwide. However, it is essential to address ethical concerns and ensure that this technology benefits all individuals, regardless of their background or location.
which utilizes deep learning algorithms to analyze dermoscopy images. Dermoscopy is a non-invasive technique that allows dermatologists to examine skin lesions with enhanced visualization, aiding in the early detection of skin cancer.
The conventional approach to skin cancer classification involves visual inspection by dermatologists, which can be subjective and prone to human error. However, with the advent of deep learning algorithms, this study presents a promising alternative that can potentially revolutionize the field of dermatology.
By training the deep learning model on a vast dataset of dermoscopy images, the algorithm is able to learn and recognize patterns and features that are indicative of different types of skin cancer. This automated approach not only reduces the reliance on subjective human interpretation but also has the potential to improve accuracy and speed in diagnosing skin cancer.
One of the key advantages of utilizing deep learning algorithms for skin cancer classification is their ability to process and analyze large amounts of data quickly. This could significantly reduce the time required for diagnosis, allowing dermatologists to provide timely treatment and improving patient outcomes.
Furthermore, this groundbreaking approach has the potential to expand access to skin cancer diagnosis in underserved areas where there may be a shortage of dermatologists. By leveraging deep learning algorithms, healthcare providers in these regions could use this technology as a screening tool, enabling earlier detection and intervention.
However, it is important to note that despite the promising results presented by this study, further research and validation are needed before implementing this approach into routine clinical practice. The dataset used for training the deep learning algorithm must be diverse and representative of various populations to ensure its generalizability.
Moreover, there are challenges associated with the interpretability of deep learning models. Understanding how the algorithm arrives at a particular classification decision can be difficult, leading to concerns about trust and transparency in clinical decision-making.
In the future, we can expect further advancements in this field, such as the integration of multiple imaging modalities or the incorporation of patient-specific data, such as demographics and medical history. Additionally, collaborations between dermatologists, computer scientists, and engineers will be crucial in refining and optimizing these deep learning algorithms for real-world clinical settings.
Overall, this study introduces an exciting and promising approach to skin cancer classification using deep learning algorithms. While there are still challenges to overcome, the potential impact on improving patient outcomes and expanding access to diagnosis is significant. Continued research and development in this area hold great promise for the future of skin cancer diagnosis and treatment.
Read the original article