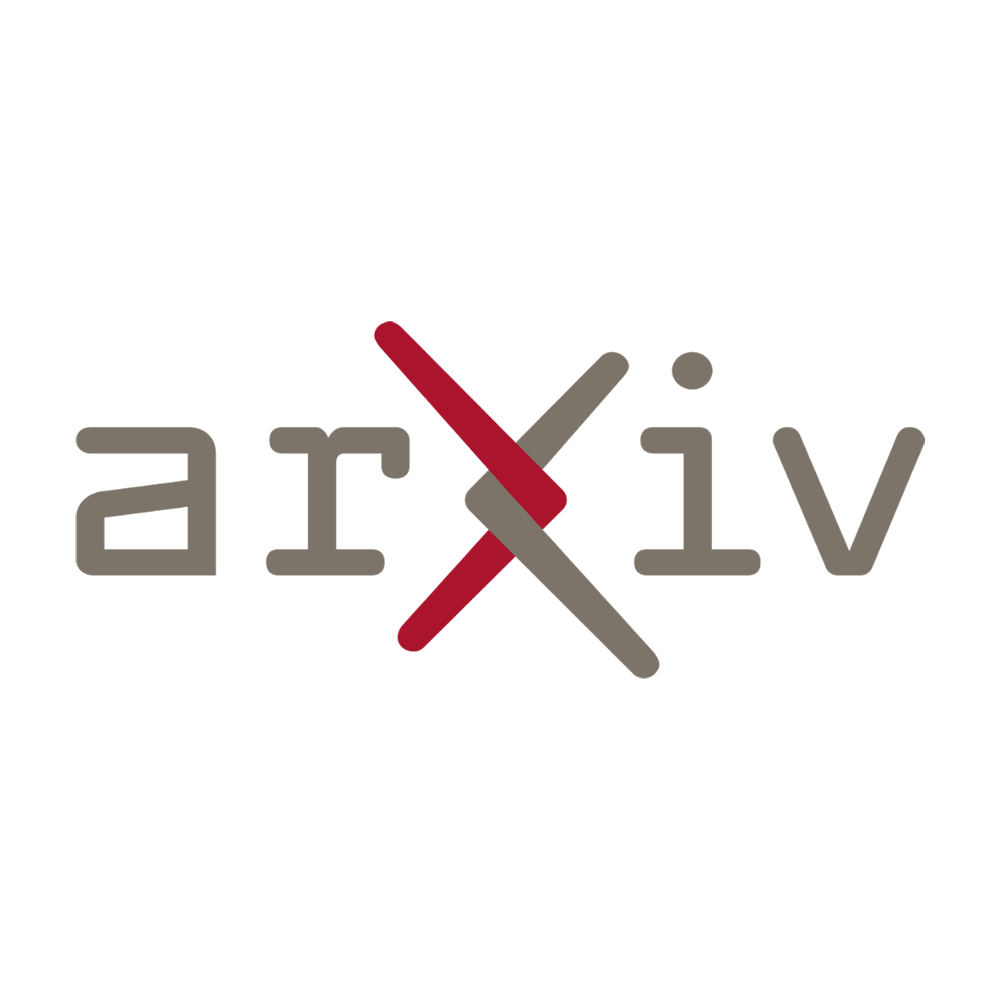
This article delves into an innovative study that explores the application of unsupervised machine learning in optimizing Profit and Loss (PnL) within the field of quantitative finance. The researchers have developed an algorithm that resembles an unsupervised version of linear regression, aiming to enhance the financial performance of various strategies. By leveraging this approach, the study presents a promising avenue for improving PnL optimization, ultimately leading to more effective decision-making and potentially higher profits in the realm of quantitative finance.
This study presents an unsupervised machine learning approach for optimizing Profit and Loss (PnL) in quantitative finance. Our algorithm, akin to an unsupervised variant of linear regression, explores the underlying themes and concepts of financial data, proposing innovative solutions and ideas for maximizing returns and minimizing risks.
Unleashing the Power of Unsupervised Machine Learning
In quantitative finance, analyzing vast amounts of data to identify patterns and make informed decisions is crucial. Traditionally, supervised machine learning techniques have been widely employed, where data is labeled and the model is trained with pre-existing outcomes. However, this approach often falls short in capturing the complex and ever-changing dynamics of financial markets.
Enter unsupervised machine learning. Unlike its supervised counterpart, unsupervised learning does not rely on labeled data. Instead, it discovers hidden structures and relationships within the data itself. By detecting patterns, correlations, and trends that escape human perception, unsupervised learning has the potential to unlock new insights and enhance decision-making processes.
The Unsupervised Variant of Linear Regression
In our study, we introduce an unsupervised variant of linear regression tailored specifically for optimizing Profit and Loss (PnL) in quantitative finance. While traditional linear regression aims to predict a target variable based on selected features, our algorithm goes a step further by extracting meaningful features directly from the data and uncovering the underlying patterns driving PnL.
By leveraging unsupervised techniques such as principal component analysis (PCA), our algorithm performs dimensionality reduction, enabling us to capture the most important information from a large number of features. It then generates a parsimonious representation of the data that allows for more accurate predictions and a deeper understanding of the factors affecting PnL.
Maximizing Returns, Minimizing Risks
Once the unsupervised variant of linear regression processes the data, it identifies the key factors that contribute to PnL optimization. By recognizing patterns and correlations, our algorithm enables traders and investment professionals to make more informed decisions, maximizing returns and minimizing risks.
Furthermore, our approach allows for the identification of potential market inefficiencies or anomalies that may have been overlooked by conventional methods. These insights can provide opportunities for strategy adjustments, portfolio rebalancing, or the exploration of new investment avenues.
Closing Thoughts
The application of unsupervised machine learning in quantitative finance opens up new possibilities for optimizing Profit and Loss (PnL) and enhancing decision-making processes. By utilizing an unsupervised variant of linear regression, we can uncover hidden patterns and relationships within financial data, leading to more accurate predictions and better risk management strategies.
“The future of quantitative finance lies in embracing innovative techniques like unsupervised machine learning. By leveraging the power of data-driven insights, we can navigate the complexities of financial markets with greater precision and agility.”
is a significant contribution to the field of quantitative finance. The use of unsupervised machine learning techniques in optimizing PnL is particularly innovative and has the potential to revolutionize the way financial institutions approach their trading strategies.
Linear regression is a widely used supervised learning technique in finance, where the relationship between independent variables and a dependent variable is modeled. However, this study takes a step further by introducing an unsupervised variant of linear regression. Unsupervised learning algorithms aim to find patterns or structure in data without being explicitly guided by labeled examples, which makes them well-suited for discovering hidden patterns in financial data.
By applying unsupervised machine learning to PnL optimization, this algorithm has the potential to uncover complex relationships and patterns that may not be easily identifiable through traditional methods. This could lead to more accurate predictions of profit and loss, ultimately enhancing trading strategies and decision-making processes.
One of the key advantages of unsupervised learning is its ability to handle large and complex datasets. In quantitative finance, where vast amounts of data are generated on a daily basis, this becomes crucial. By leveraging unsupervised learning algorithms, financial institutions can efficiently process and analyze massive datasets, improving the speed and accuracy of their decision-making processes.
Additionally, the use of unsupervised machine learning in PnL optimization opens up opportunities for developing more sophisticated trading strategies. By identifying hidden patterns and relationships in the data, financial institutions can potentially gain a competitive edge in the market. This could involve discovering new market trends, identifying trading opportunities, or even predicting market movements with greater accuracy.
However, it is important to note that the success of this approach relies heavily on the quality and relevance of the data used for training the algorithm. The study should provide insights into the data preprocessing techniques employed to ensure the algorithm’s effectiveness. Additionally, further research is needed to evaluate the algorithm’s performance on real-world financial datasets and compare it against existing approaches.
In conclusion, this study’s introduction of an unsupervised machine learning approach for optimizing PnL in quantitative finance is a significant step forward in the field. The potential to uncover hidden patterns and relationships in financial data has the power to revolutionize trading strategies and decision-making processes. Further research and experimentation are required to fully understand the algorithm’s capabilities and its practical implications in real-world financial scenarios.
Read the original article