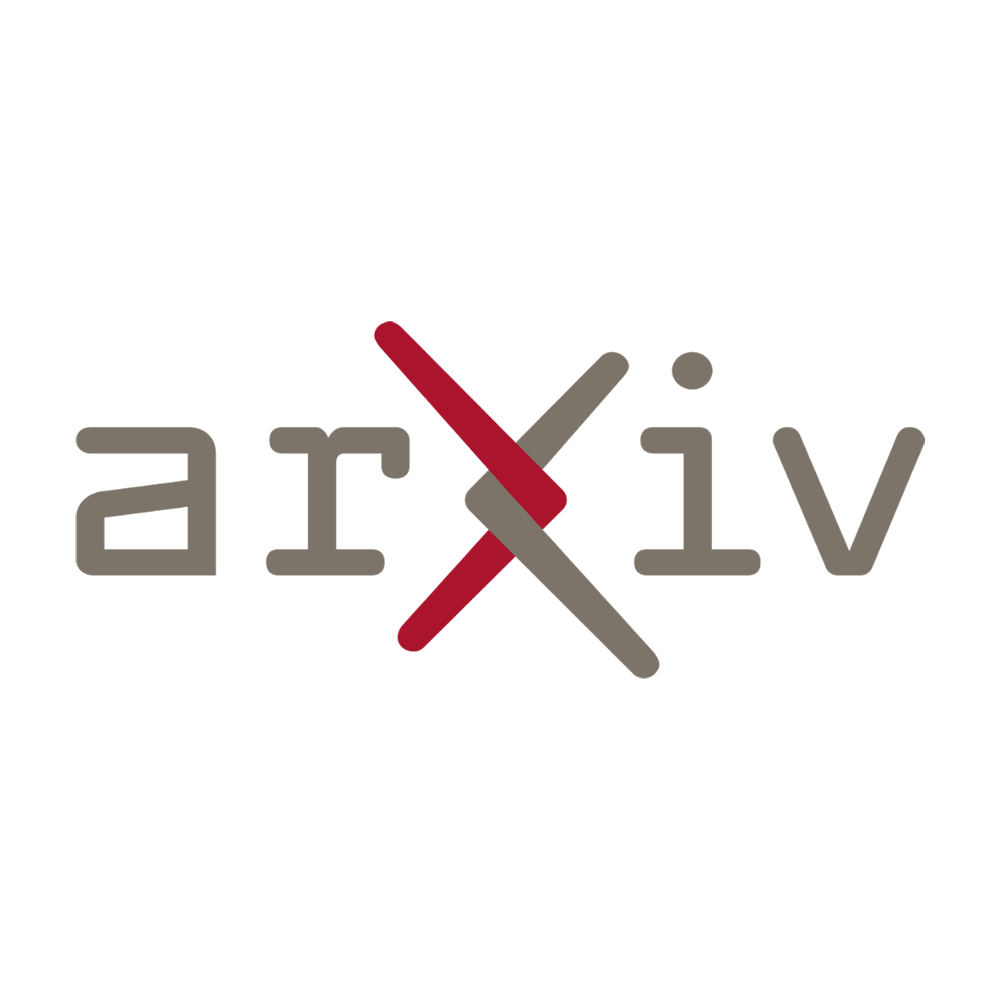
Automatic sleep staging is a crucial aspect of evaluating sleep patterns and diagnosing sleep disorders. However, current methods for sleep staging are often dependent on a single dataset and struggle to be applicable to unseen datasets. This limitation hinders the ability to effectively assess sleep and diagnose disorders across different populations. In this article, we explore the need for a more generalized approach to automatic sleep staging, highlighting the challenges faced by existing methods and the potential benefits of developing a more versatile and adaptable solution. By addressing these issues, we aim to improve the accuracy and reliability of sleep assessment, ultimately enhancing our understanding of sleep disorders and improving patient care.
Automatic sleep staging plays a crucial role in sleep assessment and disorder diagnosis. Traditional methods are often based on one particular dataset and lack the ability to be generalized to unseen datasets. The field of sleep research is constantly evolving, and new ideas and innovative solutions are needed to address these challenges.
Exploring the Underlying Themes
One underlying theme in sleep staging is the importance of data diversity. Most existing methods rely on a single dataset, which limits their generalizability. However, the human population is incredibly diverse when it comes to sleep patterns and disorders. To accurately assess sleep and diagnose disorders, we need algorithms that can handle various data sources.
Another theme is the need for interpretability and explainability in sleep staging algorithms. While deep learning models have shown impressive performance in various domains, they often suffer from a lack of interpretability. To build trust in automatic sleep staging systems, it is crucial to develop methods that can provide explanations for the decisions they make.
Innovative Solutions and Ideas
Data Augmentation and Transfer Learning
To overcome the limited generalizability of sleep staging algorithms, innovative approaches such as data augmentation and transfer learning can be employed. Data augmentation involves artificially increasing the size of the training dataset by introducing variations in existing data. By incorporating diverse data samples, algorithms can learn to handle different sleep patterns effectively.
Transfer learning, on the other hand, focuses on leveraging knowledge learned from one dataset and applying it to a different but related dataset. This approach can improve generalizability by extracting useful features from one dataset and adapting them to another. By combining data augmentation and transfer learning, we can enhance the ability of algorithms to perform well on unseen datasets.
Interpretable Deep Learning Models
Developing interpretable deep learning models specifically designed for sleep staging is another innovative solution. These models aim to combine the power of deep learning with interpretability. By incorporating attention mechanisms or visualization techniques, these models can highlight the important features or regions of input data used for decision-making. This not only provides insights into how the model arrived at a particular sleep stage prediction but also builds trust among clinicians and patients.
Conclusion
Innovations in automatic sleep staging are crucial to improve sleep assessment and disorder diagnosis. By focusing on data diversity, interpretability, and innovative techniques like data augmentation, transfer learning, and interpretable deep learning models, we can overcome the limitations of existing methods. Sleep researchers and data scientists must collaborate to develop these innovative solutions and bring them into practical use, benefiting both clinicians and individuals seeking accurate sleep evaluations.
which can limit their effectiveness and practicality in real-world applications. However, recent advancements in machine learning and artificial intelligence have shown promising results in developing automatic sleep staging algorithms that are more robust and adaptable to various datasets.
One of the key challenges in automatic sleep staging is the lack of standardized protocols across different sleep laboratories and datasets. Sleep data can vary significantly in terms of recording devices, electrode placements, and sampling rates, making it difficult to develop a one-size-fits-all approach. As a result, many existing methods struggle to generalize well when applied to unseen datasets.
To overcome this limitation, researchers have started exploring transfer learning techniques in sleep staging. Transfer learning allows models trained on one dataset to leverage their knowledge and adapt to other datasets with minimal fine-tuning. By utilizing a pre-trained model as a starting point, transfer learning can significantly improve the generalization capability of automatic sleep staging algorithms.
Another emerging trend in automatic sleep staging is the integration of multimodal data sources. Traditionally, sleep staging has relied solely on electroencephalography (EEG) signals for classification. However, recent studies have shown that combining EEG with other physiological signals such as electrooculography (EOG), electromyography (EMG), and heart rate variability (HRV) can enhance the accuracy and reliability of sleep staging algorithms. This multimodal approach provides a more comprehensive view of sleep patterns and allows for a more accurate assessment of sleep disorders.
Moving forward, it is crucial to develop standardized datasets that encompass a wide range of sleep recordings from different populations and settings. These datasets should include diverse age groups, various comorbidities, and different recording conditions to ensure the robustness and generalization of automatic sleep staging algorithms. Additionally, collaboration between sleep researchers and machine learning experts is vital to leverage the latest advancements in artificial intelligence techniques and develop more sophisticated models for sleep assessment.
In conclusion, automatic sleep staging holds great potential for improving sleep assessment and disorder diagnosis. By addressing the limitations of existing methods through transfer learning, integration of multimodal data, and standardized datasets, we can expect more accurate and adaptable algorithms in the future. These advancements will not only benefit clinical sleep medicine but also enable the development of consumer-grade sleep monitoring devices that can provide personalized insights into sleep quality and overall well-being.
Read the original article