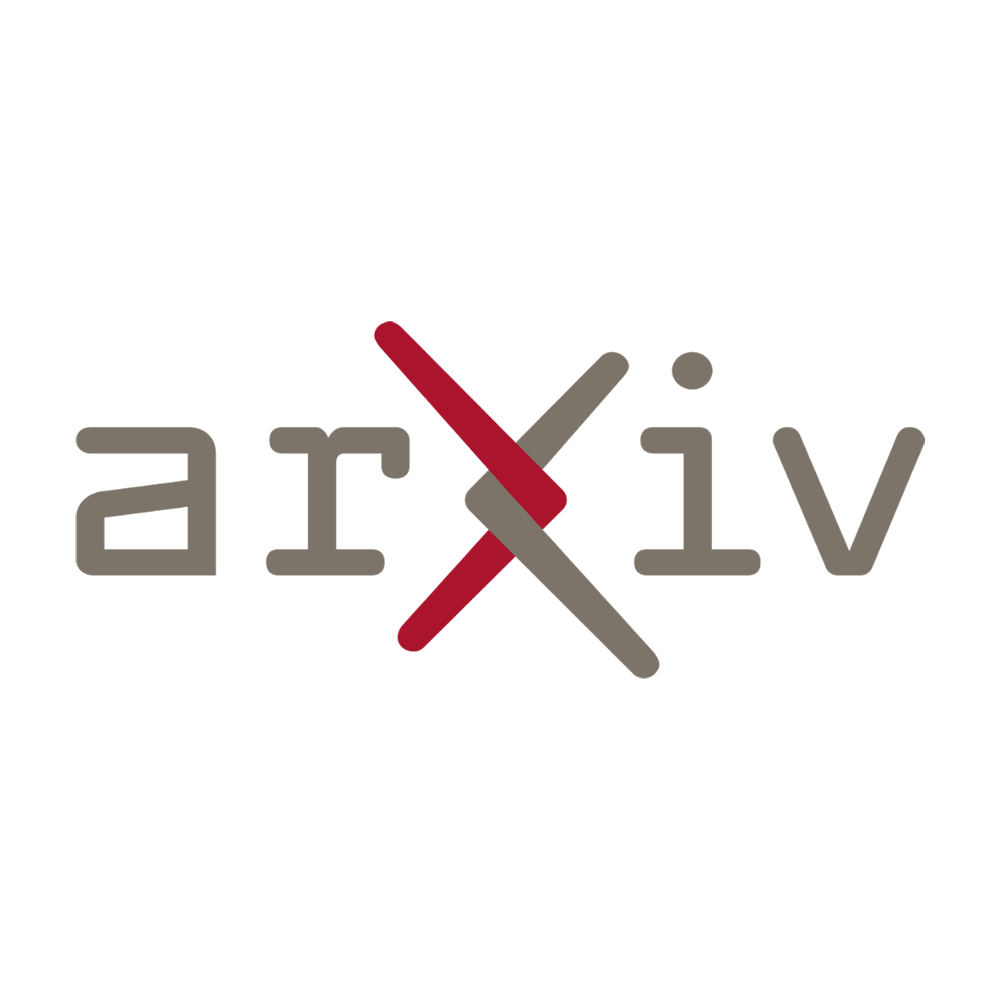
This article presents a groundbreaking framework that integrates online human state estimation, action recognition, and motion prediction to effectively address and prevent worker biomechanical risks during physical tasks. By combining these advanced technologies, this framework aims to provide early assessment and proactive measures to safeguard the well-being and safety of workers in various industries. With the potential to revolutionize workplace safety, this innovative approach offers a comprehensive solution for identifying and mitigating biomechanical risks before they lead to injuries or long-term health issues.
Exploring Innovation in Worker Biomechanical Risk Prevention
This paper proposes a framework that combines online human state estimation, action recognition, and motion prediction to enable early assessment and prevention of worker biomechanical risk during physical tasks. By examining the underlying themes and concepts of this material, we can shed light on new perspectives and propose innovative solutions.
Understanding Worker Biomechanical Risk
Worker biomechanical risk refers to the potential harm or injury posed to an individual’s musculoskeletal system due to excessive physical strain or improper body mechanics during work activities. It is essential to assess and prevent such risks to maintain the health and well-being of workers.
The Significance of Early Assessment
Early assessment plays a critical role in identifying and addressing potential biomechanical risks before they turn into severe injuries. By employing online human state estimation techniques, it becomes possible to continuously monitor and measure the physical strain experienced by workers. This real-time data can provide valuable insights into areas of concern and enable proactive prevention strategies.
Integrating Action Recognition
Action recognition algorithms can further enhance the early assessment process by identifying specific hazardous movements or postures during physical tasks. These algorithms can analyze video or sensor data to detect patterns associated with increased biomechanical risk. By alerting workers and supervisors in real-time, prompt corrective actions can be taken to mitigate potential injuries.
Motion Prediction for Injury Prevention
Incorporating motion prediction models into the framework adds an additional layer of prevention. By considering the worker’s previous movements, predictive algorithms can anticipate potential risky scenarios and provide warnings or interventions to prevent them from occurring. This proactive approach significantly reduces the chances of accidents or injuries from happening in the first place.
A Holistic Approach
The proposed framework promotes a holistic approach to worker biomechanical risk prevention. By combining online human state estimation, action recognition, and motion prediction, a comprehensive understanding of the worker’s physical condition and potential risks can be achieved. This enables targeted interventions, personalized training programs, and ergonomic improvements customized to each worker’s needs.
Innovation is key when it comes to worker safety. By leveraging cutting-edge technologies and advanced data analysis, we have the opportunity to revolutionize how we assess and prevent biomechanical risks. This not only benefits the workers themselves but also enhances productivity and creates a safer work environment for all.
Conclusion
The proposed framework provides a novel and comprehensive solution for early assessment and prevention of worker biomechanical risk. By integrating online human state estimation, action recognition, and motion prediction, companies can take substantial strides towards ensuring the health, safety, and well-being of their workers. Embracing innovation in this field will undoubtedly lead to a future where workplace injuries and their associated costs become a thing of the past.
This paper presents a highly innovative framework that aims to address the critical issue of worker biomechanical risk in industrial settings. The authors propose a comprehensive approach that combines online human state estimation, action recognition, and motion prediction to enable early assessment and prevention of such risks.
The integration of online human state estimation is a key aspect of this framework. By continuously monitoring and analyzing the physical state of workers in real-time, it provides valuable insights into their biomechanical conditions. This can include factors such as joint angles, muscle activations, and body posture. By leveraging advanced sensing technologies such as motion capture systems or wearable sensors, this framework can accurately estimate the workers’ state and detect any potential risks they may be facing.
Another important component of this framework is action recognition. By employing machine learning techniques, the system can identify and classify various actions performed by workers. This enables the system to understand the tasks being performed and the associated biomechanical demands. For example, it can differentiate between lifting heavy objects and performing repetitive motions, which are known to pose risks to workers’ health.
The framework’s ability to predict future motion patterns is a significant advancement. By considering the current state of the worker, the recognized action, and historical data, it can forecast potential future movements. This enables proactive measures to be taken to prevent biomechanical risks before they occur. For instance, if the system predicts that a worker’s posture during a specific action may lead to strain on their back, it can alert the worker or supervisor to take corrective actions or provide necessary support.
The potential applications of this framework are vast. It can be integrated into existing industrial systems to monitor worker safety and provide real-time feedback. By identifying potential risks early on, it can significantly reduce the occurrence of work-related injuries and musculoskeletal disorders. Moreover, the collected data can be used for further analysis and optimization of work processes, leading to improved efficiency and productivity.
However, there are several challenges that need to be addressed for the successful implementation of this framework. Firstly, the accuracy and reliability of the online human state estimation need to be validated across various work scenarios and environments. Robustness to occlusions, variations in lighting conditions, and different body types should be thoroughly investigated.
Additionally, the action recognition component may face challenges in accurately classifying complex and non-standard actions. The system should be trained on diverse datasets that cover a wide range of actions and work tasks to ensure its generalizability. Furthermore, the motion prediction module should consider factors such as worker fatigue, external disturbances, and unexpected events to improve its forecasting capabilities.
In conclusion, this proposed framework represents a significant advancement in the field of worker biomechanical risk assessment and prevention. By combining online human state estimation, action recognition, and motion prediction, it offers a holistic approach to early identification and mitigation of risks. With further research and development, this framework has the potential to revolutionize industrial safety practices and enhance the well-being of workers.
Read the original article