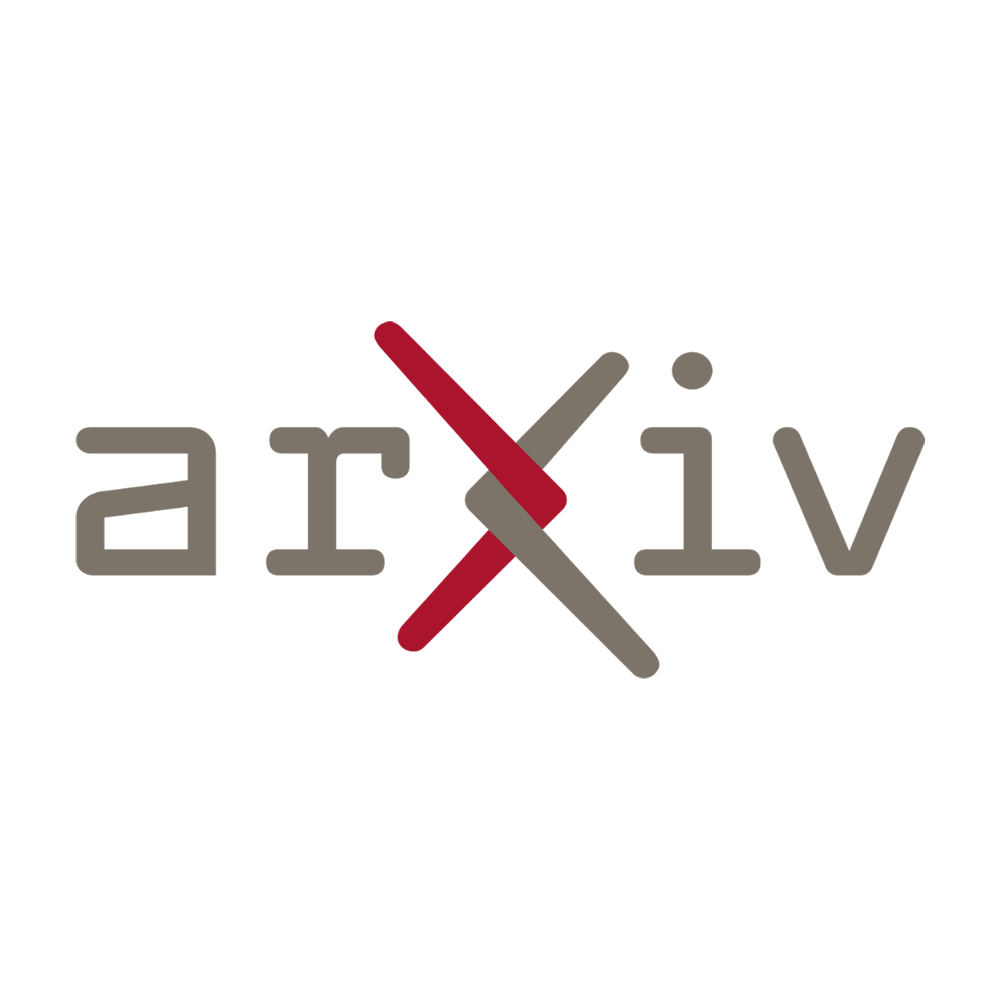
RNA secondary structure prediction is a crucial aspect of unraveling the mysteries of cellular regulation and disease mechanisms. In this article, we explore how deep learning (DL) methods have outshined traditional algorithms in accurately predicting these structures. By harnessing the power of DL, researchers have achieved remarkable advancements in understanding the intricate folding patterns of RNA molecules, paving the way for groundbreaking discoveries in various fields. Join us as we delve into the transformative potential of DL in revolutionizing RNA secondary structure prediction and its implications for unraveling the complexities of cellular processes and diseases.
Accurate RNA secondary structure prediction is vital for understanding cellular regulation and disease mechanisms. Deep learning (DL) methods have surpassed traditional algorithms by predicting complex structures more accurately. However, DL approaches often lack interpretability, making it challenging to understand the underlying biology and mechanisms driving these predictions.
In recent years, there has been a growing interest in combining DL techniques with probabilistic graphical models (PGMs) to overcome the interpretability constraint. PGMs, such as Hidden Markov Models (HMMs) and Conditional Random Fields (CRFs), offer a framework that explicitly models uncertainties and dependencies between RNA nucleotides, enabling us to capture essential biological insights.
Integrating PGMs with DL for RNA Secondary Structure Prediction
By integrating PGMs with DL, we can not only improve the accuracy of RNA secondary structure prediction but also gain valuable insights into the structural relationships and mechanisms guiding the folding process.
1. Learning interpretable features
PGMs can help us identify essential features and dependencies in RNA sequences. By training DL models in conjunction with PGMs, we can leverage the intrinsic interpretability of PGMs to guide the learning process. This integration allows us to identify biologically relevant features and understand their contributions to structure prediction.
2. Modeling uncertainties
RNA secondary structure prediction is inherently uncertain due to the experimental limitations and structural diversity within a given sequence. By incorporating HMM-based PGMs into DL models, we can explicitly model these uncertainties, providing not only accurate predictions but also confidence intervals for each nucleotide pairing. This additional information can be invaluable when studying the impact of mutations or identifying potential targets for therapeutic interventions.
3. Capturing long-range dependencies
Traditional DL approaches often struggle to capture long-range dependencies in RNA sequences, leading to suboptimal structure predictions. By combining CRFs with DL models, we can explicitly model long-range dependencies and facilitate accurate predictions of distant nucleotide pairings. These models consider the context of the entire sequence, ensuring that dependencies across distant regions are properly captured.
Potential Applications
The integration of PGMs with DL for RNA secondary structure prediction holds immense promise for various fields:
- Drug Discovery: Accurate RNA structure prediction is critical for designing small molecules that can target specific RNA structures involved in disease mechanisms. By incorporating PGMs, we can gain insights into the structural determinants of disease-relevant RNAs and identify potential drug targets.
- Gene Regulation: Understanding RNA secondary structure is crucial for deciphering gene regulation mechanisms. PGM-integrated DL models can aid in identifying structural motifs responsible for post-transcriptional regulation, shedding light on how small changes in RNA structure can affect protein synthesis and cellular processes.
- Evolutionary Biology: Studying RNA structures across different species can reveal evolutionary relationships and functional conservation. By integrating PGMs into DL models, we can uncover conserved structural elements and explore their roles in biological functions, allowing us to better understand the evolutionary dynamics of RNA molecules.
In conclusion, the combination of PGMs with DL approaches presents an innovative solution to improve RNA secondary structure prediction. This integration allows us to not only enhance prediction accuracy but also gain valuable insights into the biology and mechanisms guiding RNA folding. With applications spanning drug discovery, gene regulation, and evolutionary biology, this approach has vast potential to advance our understanding of RNA function and its impact on cellular processes and disease.
RNA secondary structure prediction is indeed crucial in unraveling the intricate mechanisms of cellular regulation and disease processes. The recent advancements in deep learning (DL) techniques have revolutionized this field by outperforming traditional algorithms in predicting accurate RNA secondary structures.
DL methods, such as recurrent neural networks (RNNs) and convolutional neural networks (CNNs), have demonstrated remarkable success in capturing complex patterns and dependencies within RNA sequences. These models excel at learning from large datasets, enabling them to recognize subtle structural motifs that might be missed by traditional algorithms.
One of the key advantages of DL-based approaches is their ability to learn directly from raw RNA sequence data, eliminating the need for handcrafted features. This data-driven approach allows DL models to capture both local and global dependencies, providing a more comprehensive understanding of RNA structure.
Moreover, DL methods can incorporate various sources of information, such as evolutionary conservation patterns, thermodynamic stability, and experimental probing data. By integrating these diverse data types, DL models can improve the accuracy of predictions and provide additional insights into the functional implications of RNA secondary structures.
Despite these remarkable achievements, challenges still remain in the field of RNA secondary structure prediction. DL models heavily rely on the availability of high-quality training data, which can be limited for certain RNA classes or species. Additionally, the interpretability of DL-based predictions can sometimes be challenging, as the models often act as black boxes, making it difficult to understand the underlying biological mechanisms.
Looking ahead, further advancements in DL techniques hold great promise for improving RNA secondary structure prediction. The integration of multi-omics data, such as RNA sequencing and structure probing experiments, could enhance the accuracy and interpretability of predictions. Additionally, the development of novel DL architectures that explicitly model physical principles governing RNA folding could provide deeper insights into the mechanisms of cellular regulation and disease progression.
In conclusion, DL methods have revolutionized RNA secondary structure prediction by surpassing traditional algorithms in accuracy and performance. Their ability to capture complex patterns and dependencies within RNA sequences has opened new avenues for understanding cellular regulation and disease mechanisms. As DL techniques continue to evolve, we can expect further breakthroughs in the field, ultimately leading to significant advancements in our understanding of RNA biology and its implications for human health.
Read the original article