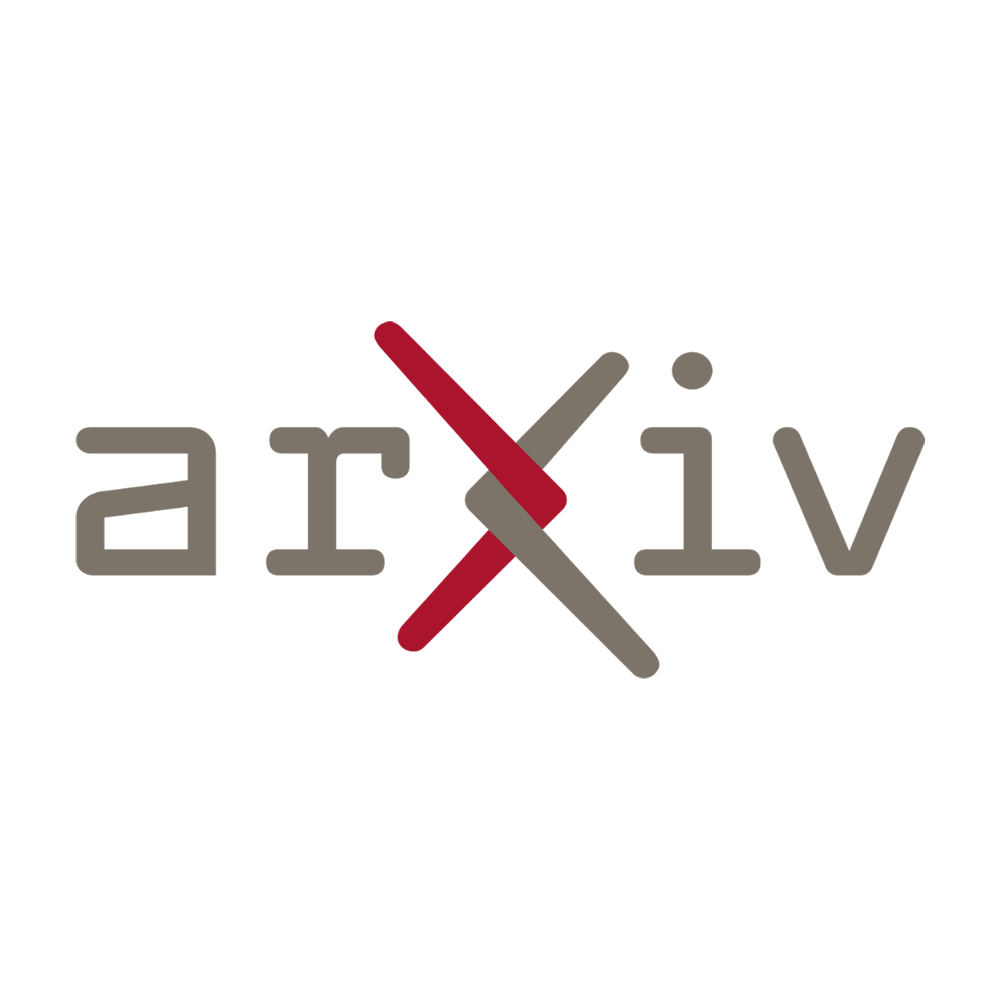
Spectral lightcurves have proven to be a powerful tool in determining the attitude and rotation of spacecraft. By analyzing time series single-pixel spectral measurements, researchers have developed two distinct methods to extract valuable information about the spacecraft’s orientation. One method relies on…
Spectral lightcurves are a valuable tool in determining the attitude and rotation of spacecraft. By analyzing time series single-pixel spectral measurements, scientists can gain insights into the behavior and positioning of these celestial vehicles. However, current methods have limitations and can be improved upon to enhance accuracy and efficiency.
The Power of Spectral Lightcurves
Spectral lightcurves provide a wealth of information about a spacecraft’s attitude and rotation. By observing the changes in spectral measurements over time, scientists can detect patterns and anomalies that indicate movement or rotation. This data is crucial for understanding the behavior and dynamics of spacecraft, especially in complex missions or long-duration space travel.
The Limitations of Current Methods
Currently, two methods are commonly used to analyze spectral lightcurves. One method involves directly fitting the spectral data to a model, while the other relies on cross-correlation techniques. While these methods have proven useful, they both have limitations that hinder their accuracy and efficiency.
In the direct fitting method, the spectral data is compared to various predefined models to find the best match. However, this approach is time-consuming and requires significant computational resources. Additionally, it assumes a perfect understanding of the spectral behavior, which may not always hold true in real-world scenarios.
The cross-correlation method, on the other hand, compares the spectral data with a reference lightcurve to determine similarity. While this technique is faster than direct fitting, it relies heavily on the quality of the reference lightcurve. If the reference is not representative of the spacecraft’s true behavior, the results may be inaccurate.
Innovative Solutions for Enhanced Analysis
To overcome these limitations and improve the analysis of spectral lightcurves, innovative solutions can be proposed. One approach is to combine machine learning algorithms with spectral analysis techniques.
By training machine learning models on a large dataset of spectral lightcurves, these algorithms can learn patterns and behaviors that may not be easily detectable to the human eye. This would enhance the accuracy of identifying rotational movements and provide a more comprehensive understanding of spacecraft dynamics.
Another innovative solution is to develop adaptive spectral models that can adjust and refine themselves based on real-time measurements. These models would continuously learn and update their understanding of the spacecraft’s spectral behavior, leading to more accurate analysis and predictions.
The Benefits of Innovative Solutions
Implementing these innovative solutions would bring several benefits to the field of spacecraft analysis. Firstly, the combination of machine learning algorithms and spectral analysis techniques would save significant time and computational resources by automating the analysis process.
Secondly, the use of adaptive spectral models would enable real-time monitoring and adjustment. This would be particularly valuable in dynamic situations where a spacecraft’s behavior may change rapidly. By constantly updating their understanding, these models would provide accurate and up-to-date information, ensuring the safety and efficiency of space missions.
“Enhancing the analysis of spectral lightcurves is essential for advancing our knowledge of spacecraft dynamics and improving mission performance.”
In Conclusion
Spectral lightcurves play a crucial role in inferring the attitude and rotation of spacecraft. While current methods are valuable, they have limitations that can be overcome through innovative solutions.
By combining machine learning algorithms with spectral analysis techniques and developing adaptive spectral models, scientists can enhance the accuracy and efficiency of spacecraft analysis. These advancements will provide valuable insights into spacecraft dynamics, improving mission performance, and driving further advancements in space exploration.
the analysis of the spectral content, and the other based on the analysis of the temporal variations in the lightcurves.
The first method, which analyzes the spectral content, involves examining the intensity of light at different wavelengths. By studying the variations in intensity, scientists can deduce information about the spacecraft’s attitude and rotation. This method relies on the fact that different orientations of the spacecraft will result in varying amounts of light being observed at different wavelengths. For example, if a spacecraft is rotating, the observed intensity at a particular wavelength will change periodically as different parts of the spacecraft come into view.
To extract meaningful information from these spectral measurements, sophisticated algorithms and statistical techniques are employed. These algorithms analyze the patterns of intensity variations at different wavelengths to determine the spacecraft’s attitude and rotation parameters. By comparing the observed spectral lightcurve with known models and simulations, scientists can accurately infer the spacecraft’s orientation and motion.
The second method focuses on analyzing the temporal variations in the lightcurves. Instead of examining the spectral content, this approach looks at how the intensity of light changes over time. By studying these temporal variations, scientists can gain insights into the spacecraft’s attitude and rotation.
This method involves analyzing the periodicity, amplitude, and shape of the lightcurves. The periodicity can reveal the spacecraft’s rotational period, while the amplitude and shape provide information about its attitude. For example, a larger amplitude might indicate a more pronounced rotation or a change in attitude.
Similar to the spectral analysis method, advanced algorithms and statistical techniques are employed to interpret these temporal variations. These algorithms search for patterns and correlations in the lightcurves to infer the spacecraft’s orientation and motion accurately.
Both methods have their strengths and limitations. The spectral analysis method provides detailed information about the spacecraft’s orientation at different wavelengths, allowing for a more comprehensive understanding of its behavior. On the other hand, the temporal analysis method provides insights into the dynamic changes in attitude and rotation over time.
Looking ahead, advancements in instrumentation and data analysis techniques will likely enhance the accuracy and resolution of these methods. Future spacecraft missions may incorporate more advanced sensors capable of capturing high-resolution spectral measurements, enabling even more precise determination of attitude and rotation. Additionally, machine learning algorithms could be employed to improve the analysis of spectral lightcurves, allowing for faster and more automated processing of the data.
Furthermore, combining the strengths of both spectral and temporal analysis methods could lead to more robust and accurate spacecraft attitude and rotation determination. By integrating the information obtained from both approaches, scientists can mitigate the limitations of each method and obtain a more comprehensive understanding of the spacecraft’s dynamics.
In conclusion, spectral lightcurves provide valuable insights into a spacecraft’s attitude and rotation. By analyzing the spectral content and temporal variations in the lightcurves, scientists can infer critical parameters that characterize the spacecraft’s orientation and motion. Continued advancements in technology and analysis techniques will likely drive further improvements in our ability to accurately determine spacecraft attitudes and rotations, paving the way for enhanced mission planning, navigation, and control.
Read the original article