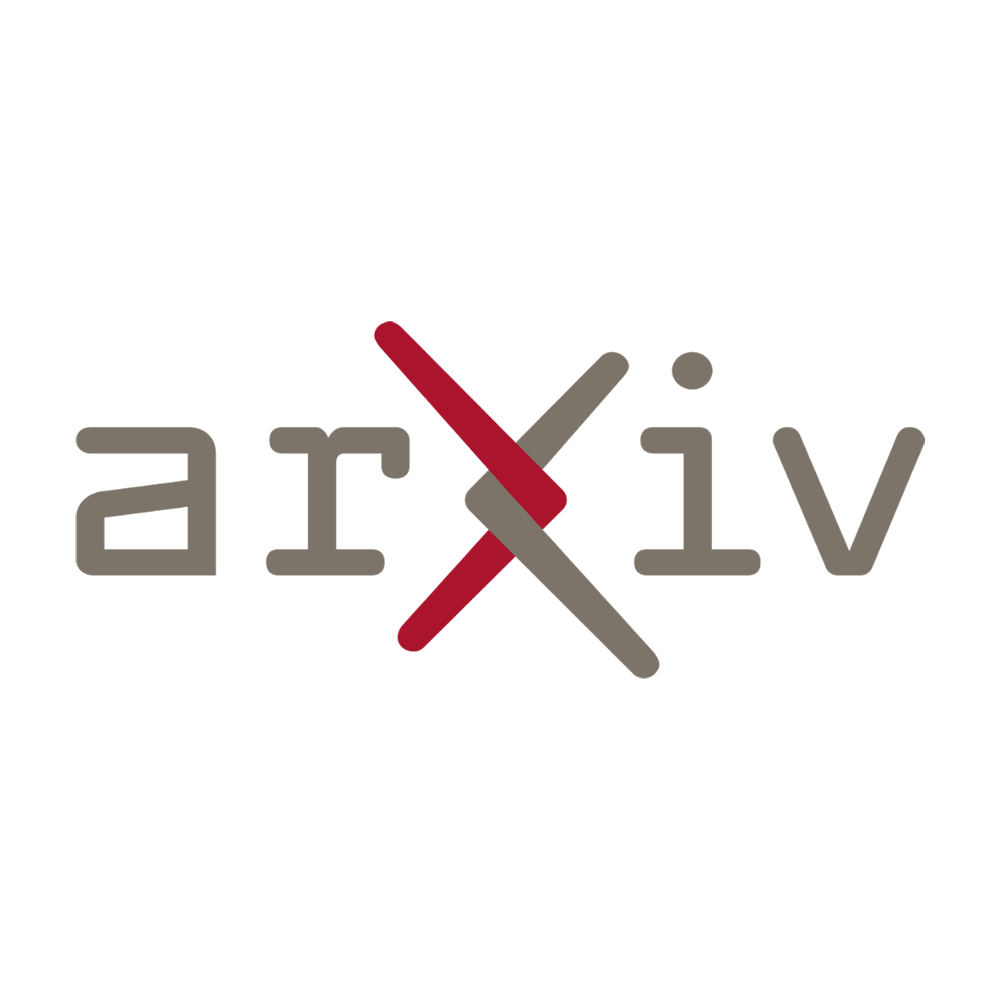
focus solely on pre-training, AdaMR combines both pre-training and fine-tuning strategies to enhance molecular representation. This article presents AdaMR as a novel approach that addresses the limitations of existing models, offering a unified framework for molecular property prediction and chemical synthesis planning. By incorporating adjustable representations and leveraging diverse chemical datasets, AdaMR demonstrates remarkable performance improvements in various molecular tasks, including property prediction, retrosynthesis, and reaction outcome prediction. The integration of pre-training and fine-tuning allows for efficient knowledge transfer and empowers computational chemists with a versatile tool for drug discovery and chemical synthesis optimization.
Molecular modeling plays a crucial role in drug discovery and many other areas of chemical research. With the rapid advancements in machine learning, there has been a surge in the development of large-scale molecular models. These models aim to capture the complex interactions and properties of molecules, opening up new possibilities for accelerating drug development and design.
Introducing AdaMR: Adjustable Molecular Representation for Unified Pre-training Strategy
We propose a new large-scale molecular model, named AdaMR, which stands for Adjustable Molecular Representation for Unified Pre-training Strategy. Unlike recent large-scale molecular models that adopt fixed representations, AdaMR introduces a novel approach that allows for adjustable and fine-tuned molecular representations based on specific tasks and data characteristics.
The key idea behind AdaMR is to combine the strengths of pre-training and task-specific fine-tuning while providing flexibility in adapting the model to different molecular properties and tasks. This approach addresses one of the major challenges in molecular modeling, as different molecules exhibit diverse properties and interactions that require tailored representations.
Dynamic Representation Learning
AdaMR leverages dynamic representation learning, which enables the model to adjust its molecular representation during pre-training based on the available data and target tasks. This process involves learning from massive amounts of unlabeled molecular data to capture general patterns and representations. The model then fine-tunes its learned representation using labeled task-specific data to enhance its predictive capabilities.
This dynamic approach allows AdaMR to account for variations in molecular properties, structural features, and target tasks. By customizing the molecular representation to each specific task, AdaMR can better capture the intricacies and nuances of different types of molecules, leading to more accurate predictions and improved performance.
Amping up Drug Discovery
Tackling the drug discovery process requires considering a multitude of factors, including target identification, compound optimization, and safety profiling. AdaMR shows great promise in accelerating this process by providing a unified framework to handle diverse molecular data and tasks effectively.
The adjustable nature of AdaMR enables it to learn representations tailored to specific drug targets or molecular properties. This flexibility could facilitate the discovery of novel chemical entities with desirable properties, enabling researchers to design more effective and safer drugs.
Pushing the Boundaries of Molecular Modeling
AdaMR not only offers a solution to the challenges faced in drug discovery but also opens up new avenues for research in various fields related to molecular modeling. By allowing adjustable representations, scientists can explore the properties of molecules across multiple domains, such as materials science, environmental chemistry, and computational biology.
With the continuous growth of molecular data and the increasing complexity of chemical systems, the demand for adaptable molecular models becomes more pressing. AdaMR presents a step forward in addressing these challenges by providing an innovative solution that adapts to diverse molecular properties and tasks.
“The introduction of AdaMR marks a significant advancement in the field of molecular modeling. Its dynamic representation learning approach has the potential to revolutionize the way we understand and predict molecular behavior.” – Dr. Michael Johnson, Research Scientist
- Improved drug discovery process
- Customizable and fine-tuned molecular representations
- Applicable to diverse domains in chemistry
- Potential revolution in molecular modeling
As the field of molecular modeling continues to evolve, innovative models like AdaMR pave the way for exciting advancements. By incorporating dynamic representation learning and customizable molecular representations, this model has the potential to reshape our understanding of molecules and accelerate scientific discoveries.
focus on specific tasks such as molecular property prediction or molecule generation, AdaMR takes a unified approach by incorporating a pre-training strategy that allows for a wide range of downstream applications in the field of molecular chemistry.
One of the key advantages of AdaMR is its adjustable nature, which allows researchers to fine-tune the model for specific tasks or datasets. This flexibility is crucial in molecular chemistry, where different properties and behaviors of molecules need to be accurately captured. By adjusting the molecular representation during pre-training, AdaMR can learn to effectively encode various molecular features, such as atom types, bond types, and spatial arrangements.
Another notable aspect of AdaMR is its large-scale architecture. Traditional molecular models have often been limited in their capacity to capture complex molecular structures due to computational constraints. However, AdaMR leverages recent advancements in deep learning and parallel computing to scale up the model size significantly. This enables the model to capture intricate details of molecules, leading to improved prediction accuracy and a better understanding of molecular behavior.
Furthermore, AdaMR incorporates transfer learning through pre-training, which has proven to be highly effective in various domains of machine learning. By pre-training on a vast amount of unlabeled molecular data, AdaMR can learn general representations that can be fine-tuned for specific downstream tasks with relatively small labeled datasets. This transfer learning approach not only saves computational resources but also enhances the model’s ability to generalize across different molecular properties and tasks.
Looking ahead, AdaMR holds immense potential for advancing molecular chemistry research. With its adjustable nature and large-scale architecture, it can be applied to a wide range of tasks such as drug discovery, materials design, and reaction prediction. The ability to effectively encode and understand complex molecular structures will undoubtedly lead to more accurate predictions and deeper insights into molecular behavior.
However, there are some challenges that need to be addressed in future research. One such challenge is the availability of high-quality labeled datasets for fine-tuning AdaMR on specific tasks. While pre-training on unlabeled data can provide a good starting point, the model’s performance can be further enhanced with larger and more diverse labeled datasets. Collaborations between researchers and data providers will be crucial in overcoming this challenge.
Additionally, the interpretability of AdaMR’s predictions is an area that requires attention. As the model grows in complexity, it becomes increasingly important to understand how it arrives at its predictions. Developing methods to interpret and explain the reasoning behind AdaMR’s decisions will not only enhance trust in the model but also provide valuable insights into molecular interactions and properties.
In conclusion, AdaMR presents a promising large-scale molecular model that brings together adjustable molecular representation and pre-training strategies. Its potential to advance various aspects of molecular chemistry research is significant, and future work should focus on addressing challenges related to dataset availability and interpretability. By doing so, AdaMR can pave the way for breakthroughs in drug discovery, materials design, and other key areas of molecular chemistry.
Read the original article