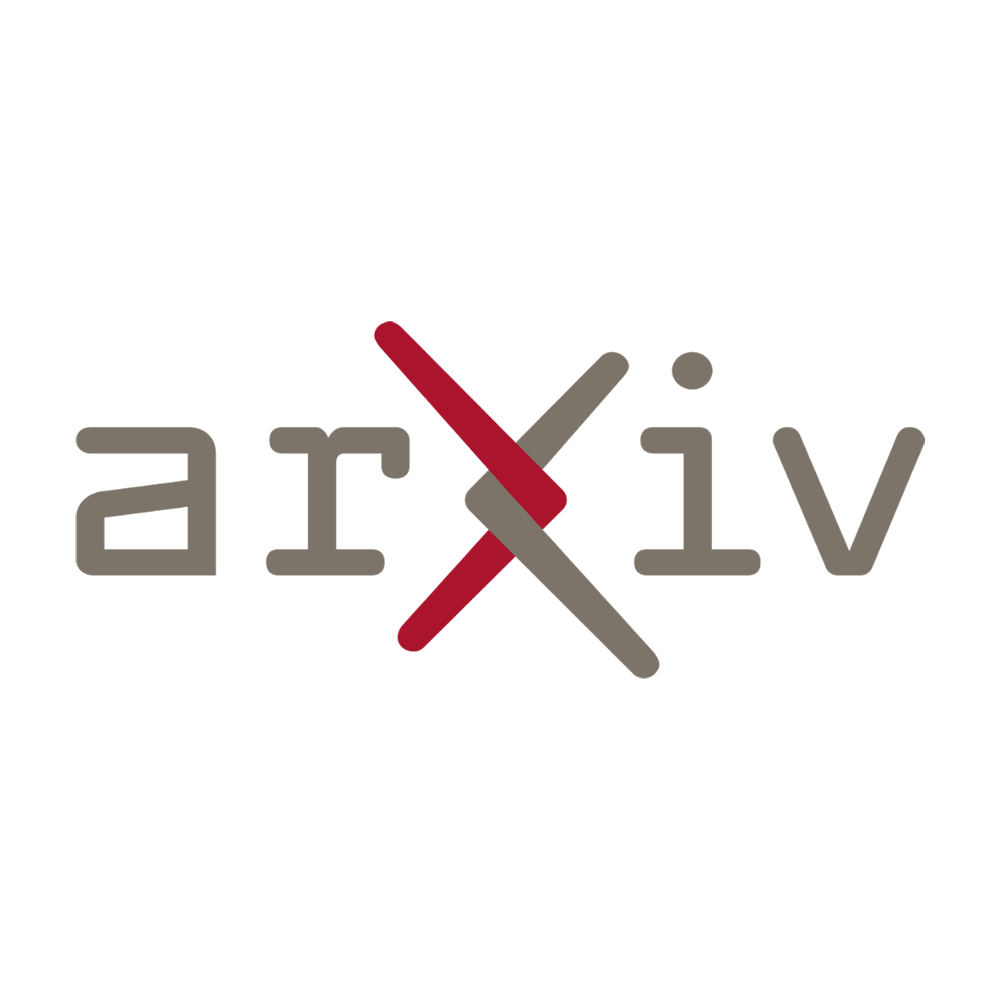
are addressed in the context of curve-based design sketches. The article explores a novel approach to style transfer, focusing on preserving the unique characteristics and aesthetics of hand-drawn curves. By combining deep learning techniques and parametric modeling, the research aims to overcome the limitations of existing methods and provide a more efficient and accurate framework for transforming design sketches into stylized artworks. This article sheds light on the potential applications of curve-based style transfer in various creative industries, such as architecture, fashion, and graphic design, offering a promising avenue for artists and designers to explore new possibilities in their work.
A Paradigm Shift in Curve-Based Design Sketch Style Transfer
In recent years, neural style transfer has emerged as a powerful technique for transforming images, allowing artists and designers to apply the style of one image to another. However, existing frameworks primarily focus on transferring the style of photographs or paintings, leaving a significant gap in the world of curve-based design sketches. This is where a groundbreaking new research presents a fresh approach to parametric style transfer, catering specifically to curve-based design sketches.
The Traditional Challenges
Curve-based design sketches have long been an integral part of the creative process for artists and designers. These sketches represent the underlying structure and essence of the final design, providing a guiding framework for the subsequent stages. However, when it comes to transferring styles between these sketches, traditional challenges arise due to their unique characteristics.
Unlike photographs or paintings, curve-based design sketches have no precise color information or pixel-level details. They rely on the simplicity and elegance of curves to convey their meaning. This simplicity makes it difficult for existing neural style transfer algorithms to accurately capture and transform the style of these sketches.
Furthermore, curve-based design sketches often contain intricate and interconnected elements that contribute to their overall aesthetic. Existing style transfer frameworks struggle to preserve these intricate relationships, leading to distorted and unsatisfactory results.
A New Framework for Curve-Based Design Sketch Style Transfer
The research introduces a novel parametric style transfer framework explicitly tailored to the unique qualities of curve-based design sketches. This framework utilizes a combination of deep learning techniques and mathematical modeling to overcome the challenges posed by curve-based sketches.
Instead of relying solely on pixel-level details, the framework focuses on capturing the underlying structure of the sketches. It treats curves as the fundamental basis for style transfer, allowing for more precise and faithful transformations. By understanding the role of curves in conveying style, this new approach delivers visually compelling results that retain the essence of the original sketch.
Moreover, the framework incorporates advanced graph-based algorithms to preserve the intricate relationships between elements within the sketches. By analyzing the connectivity and hierarchy of curves, the framework ensures that style transfer does not disrupt the overall aesthetic balance and coherence of the design.
Innovative Applications and Future Possibilities
This groundbreaking research opens up a world of possibilities for artists, designers, and creative professionals. With this new parametric style transfer framework, curve-based design sketches can now be seamlessly transformed, allowing for experimentation, exploration, and innovation in various design domains.
One exciting application is the ability to transfer the style of iconic design sketches from renowned artists and designers onto one’s own work. This not only pays homage to the masters but also offers a unique opportunity to incorporate their distinct styles into contemporary designs.
Furthermore, this framework paves the way for collaborative design processes. Design teams can now easily explore different stylistic variations, iterate on design concepts, and seamlessly merge their ideas by transferring styles between sketches. Such collaborative approaches empower designers to create more dynamic and cohesive visual narratives.
In conclusion, this research breakthrough presents a paradigm shift in curve-based design sketch style transfer. By addressing the traditional challenges and providing innovative solutions, the new parametric style transfer framework unlocks the potential of curve-based design sketches, fostering creativity, collaboration, and pushing the boundaries of design.
“With this new framework, curve-based design sketches become a canvas for artistic exploration and innovation.”
such as preserving global structure and fine details, are addressed by focusing on curve-based design sketches. The proposed parametric style transfer framework leverages the inherent characteristics of curves, allowing for more control over the style transfer process.
One of the main advantages of this approach is that it enables designers to create unique and customized styles for their sketches. By manipulating the parameters of the curves, they can easily adjust the level of abstraction, stroke thickness, and other stylistic elements. This flexibility opens up new possibilities for artists and designers to express their creativity and produce visually appealing designs.
Moreover, the researchers have introduced a novel loss function that takes into account both the global structure and local details of the design sketches. This loss function helps to maintain the overall shape and proportions of the original sketch while incorporating the desired style. By striking a balance between these two aspects, the framework ensures that the style transfer is faithful to the original sketch while still incorporating the desired artistic style.
However, it is important to note that there are some limitations to this framework. Since it focuses on curve-based design sketches, it may not be suitable for other types of images or designs. Additionally, the proposed framework heavily relies on the quality of the input sketches. If the initial sketch lacks clarity or coherence, it may impact the effectiveness of the style transfer process.
Looking ahead, there are several potential directions for further research in this area. One interesting avenue could be exploring how this parametric style transfer framework could be extended to incorporate other design elements such as color or texture. By considering multiple design dimensions, it would be possible to create even more diverse and visually captivating results.
Another area that could benefit from future research is the development of more advanced optimization algorithms to enhance the efficiency and speed of the style transfer process. As neural networks become more complex and demanding, finding ways to optimize their performance is crucial. This could involve exploring techniques such as parallel computing or leveraging hardware acceleration to speed up the style transfer process.
Overall, this research presents an exciting development in the field of style transfer, specifically tailored for curve-based design sketches. By addressing traditional challenges and introducing a parametric framework, it offers designers and artists a powerful tool to create unique and visually stunning designs. With further advancements and refinements, this framework has the potential to revolutionize the way we approach style transfer in the design industry.
Read the original article