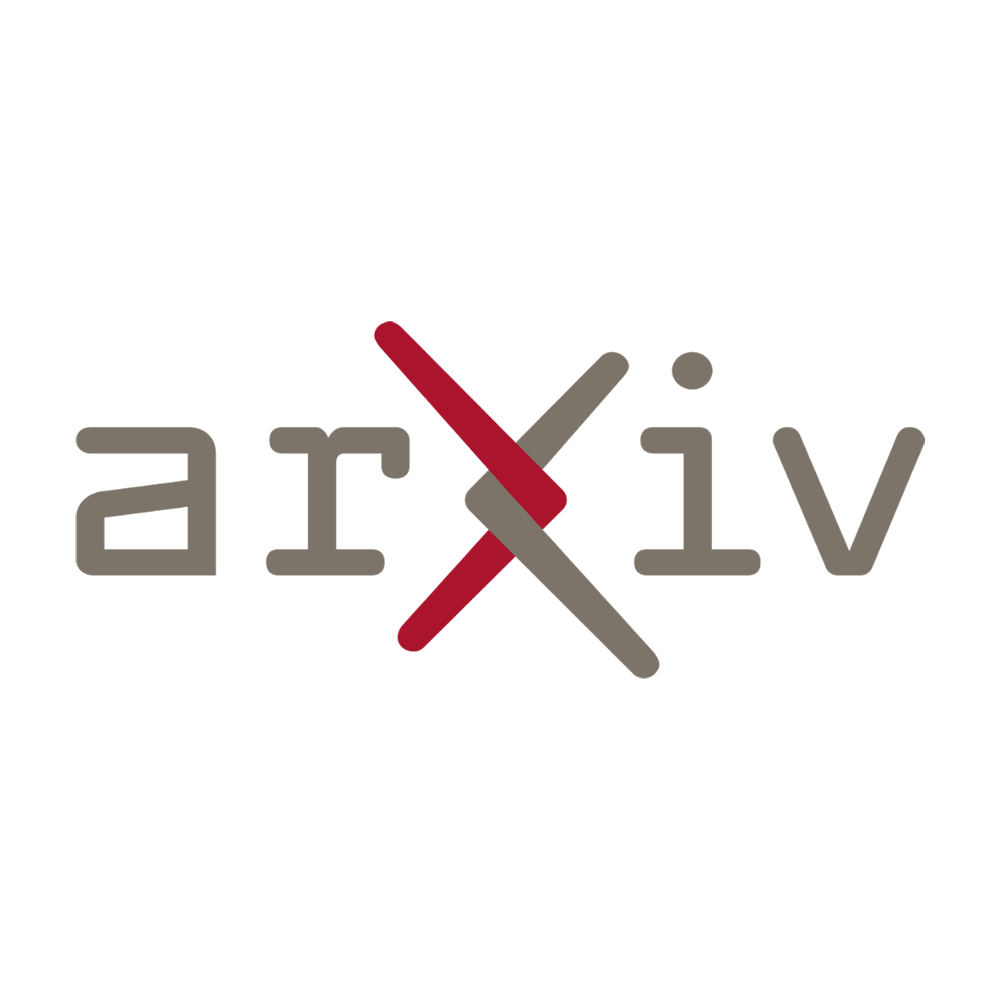
have been developed to predict the binding affinity between T-cell receptors (TCRs) and epitopes, but their accuracy remains a challenge. In a recent study, researchers aimed to improve the accuracy of binding affinity prediction by combining multiple computational methods. By integrating machine learning algorithms and structural modeling techniques, they were able to achieve significant improvements in predicting TCR-epitope binding affinities. This breakthrough has important implications for the development of effective immunotherapy strategies, as accurate binding affinity prediction can help identify potential targets for TCR-based therapies and optimize treatment outcomes.
An Innovative Approach to Predicting Binding Affinities in Immunotherapy
An accurate binding affinity prediction between T-cell receptors and epitopes contributes decisively to developing successful immunotherapy strategies. While some state-of-the-art computational methods have been developed to address this challenge, there is still a need for innovative approaches that can further improve the accuracy and efficiency of these predictions.
Understanding the Importance of Binding Affinity Prediction
In the field of immunotherapy, the binding affinity between T-cell receptors (TCRs) and epitopes plays a crucial role in determining the effectiveness of treatments. It is the strength of this interaction that dictates whether T-cells will recognize and effectively attack specific cancer cells or pathogens.
Accurate prediction of binding affinities is essential for several reasons. Firstly, it helps in the identification of potential immunotherapy targets by quickly screening large databases of epitopes and selecting those with a high predicted affinity for specific TCRs. This saves researchers time and resources by narrowing down the search space.
Secondly, predicting binding affinities allows for the design of novel immunotherapies tailored to individual patients. By assessing the affinity between a patient’s TCR repertoire and possible treatment targets, personalized therapies can be developed to enhance efficacy and minimize side effects.
The Current State of Computational Methods
Currently, several computational methods exist to predict TCR-epitope binding affinities. These methods often rely on machine learning algorithms trained on experimental binding data. While they have achieved moderate success, there are challenges that limit their accuracy and applicability.
One challenge is the scarcity of reliable and diverse training data. Experimental assays to measure binding affinities are time-consuming and expensive, resulting in limited data availability. This scarcity restricts the ability of algorithms to learn the full complexity of TCR-epitope interactions and hinders their predictive accuracy.
Another challenge is the inherent sequence variability of TCRs and epitopes. The binding affinity between a TCR and an epitope is influenced by multiple factors, including specific amino acid residues. However, existing methods often struggle to capture the subtle nuances of these interactions due to limitations in encoding and representation.
Proposing an Innovative Solution
To address these challenges and improve the accuracy and efficiency of binding affinity prediction, we propose an innovative approach that combines deep learning techniques with advanced bioinformatics strategies.
In our proposed approach, we leverage the power of deep learning models, such as recurrent neural networks or transformer-based architectures, to learn the complex patterns and dependencies within TCR-epitope sequences. These models can capture both local and global interactions, enabling more accurate predictions even in cases with limited training data.
Furthermore, we incorporate advanced bioinformatic techniques to enhance the encoding and representation of TCR-epitope sequences. By incorporating structural information, physicochemical properties, and evolutionary profiles, we aim to capture the intricate details that contribute to binding affinity.
The Potential Impact
If successful, our innovative approach could revolutionize the field of immunotherapy by providing researchers and clinicians with a highly accurate and efficient method for predicting TCR-epitope binding affinities. This could greatly expedite the discovery of novel immunotherapy targets, accelerate the development of personalized treatments, and ultimately improve patient outcomes.
In conclusion, accurately predicting binding affinities between T-cell receptors and epitopes is crucial for the success of immunotherapy. While existing methods have shown promise, an innovative combination of deep learning and bioinformatics techniques could significantly enhance the accuracy and efficiency of these predictions. By overcoming the limitations of current approaches, we can pave the way for more effective and personalized immunotherapies.
have been developed to predict the binding affinity between T-cell receptors (TCRs) and their target epitopes. These methods play a crucial role in advancing the field of immunotherapy by aiding in the design of effective treatments for various diseases, including cancer.
One important aspect of immunotherapy is the ability to identify and select TCRs that have a high affinity for specific epitopes. This is because the binding affinity between a TCR and its target epitope determines the strength of the immune response and ultimately influences the success of the therapy. Accurate predictions of binding affinity enable researchers to prioritize and select TCRs with the highest potential for therapeutic efficacy.
State-of-the-art computational methods for predicting TCR-epitope binding affinity utilize machine learning algorithms, such as support vector machines (SVMs) and deep neural networks (DNNs). These algorithms are trained on large datasets of known TCR-epitope interactions, which allows them to learn patterns and features that contribute to binding affinity. By leveraging these learned patterns, these computational methods can then predict binding affinities for new TCR-epitope pairs.
One key advantage of these computational methods is their ability to handle the vast diversity of TCR sequences and epitope structures. TCRs are highly diverse, with millions of possible combinations, and each TCR has unique binding preferences. Additionally, epitopes can have various structural features that influence their interaction with TCRs. By considering these complexities, computational methods provide a more comprehensive understanding of TCR-epitope interactions than traditional experimental approaches alone.
However, despite the advancements made in computational prediction methods, there are still challenges to overcome. One major challenge is the limited availability of high-quality training data. Obtaining experimental binding affinity measurements for a wide range of TCR-epitope pairs is a time-consuming and costly process. As a result, training datasets may be biased or incomplete, leading to potential limitations in the accuracy and generalizability of the predictive models.
To address these challenges, researchers are exploring strategies to improve the training data quality and quantity. This includes efforts to generate large-scale experimental datasets through high-throughput technologies and the integration of diverse data sources, such as structural information and evolutionary conservation. Additionally, advancements in data augmentation techniques and transfer learning approaches hold promise in enhancing the predictive capabilities of computational models.
Looking ahead, the future of accurate binding affinity prediction between TCRs and epitopes lies in the integration of multiple data types and the development of more sophisticated machine learning algorithms. By combining structural, sequence, and functional information, researchers can gain a deeper understanding of TCR-epitope interactions and improve the accuracy of binding affinity predictions. Furthermore, advancements in interpretability and explainability of computational models will enable researchers to uncover the underlying mechanisms driving TCR-epitope recognition, leading to the design of even more effective immunotherapies.
In conclusion, accurate binding affinity prediction is a critical component in the development of successful immunotherapy strategies. State-of-the-art computational methods have made significant progress in this field, leveraging machine learning algorithms and diverse datasets. However, challenges still exist, particularly in obtaining high-quality training data. Future advancements in data generation, integration, and algorithm development will further enhance our ability to predict TCR-epitope binding affinities, ultimately leading to more precise and personalized immunotherapies for patients.
Read the original article