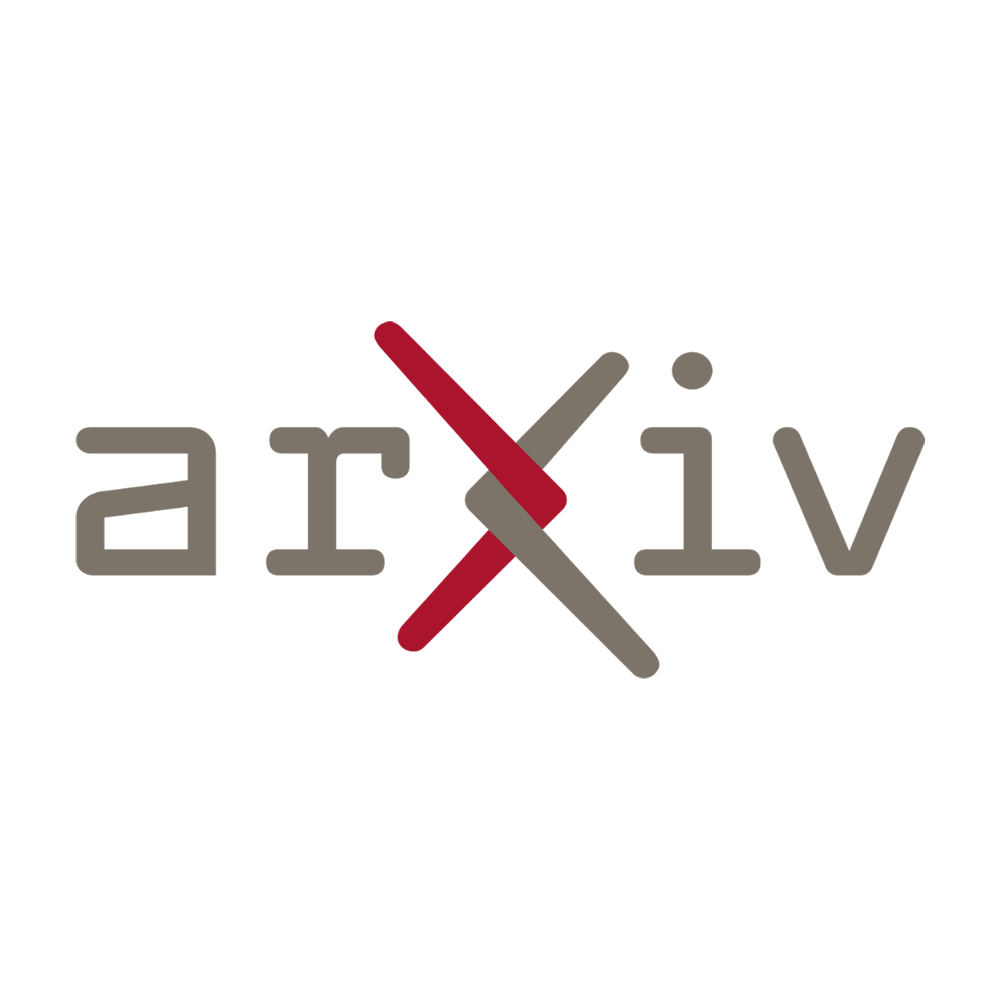
In a groundbreaking study, researchers have discovered a fascinating connection between the temporal patterns of geospatial activity and the type of land use. This correlation opens up new possibilities for understanding and analyzing landscapes in a more comprehensive way. To delve deeper into this relationship, a novel self-supervised approach has been developed, which aims to stratify landscapes based on the time spent engaging in mobility activities. By employing this innovative method, researchers hope to gain valuable insights into how different areas are utilized and potentially uncover hidden patterns that can inform urban planning and resource allocation decisions. This article explores the core themes of this study, highlighting the significance of geospatial activity temporal patterns in understanding land use dynamics and the potential implications for future research and practical applications.
Understanding the Relationship Between Geospatial Activity Patterns and Land Use
In the ever-evolving field of geospatial analysis, researchers have long sought to uncover the intricate relationship between human activity patterns and the types of land use in a given area. By understanding this correlation, it becomes possible to develop innovative solutions and ideas for urban planning, transportation management, and environmental conservation.
Introducing a Novel Self-Supervised Approach
A new and exciting approach to this complex issue comes in the form of a self-supervised method for stratifying landscapes based on mobility activity time. This innovative solution brings together advanced data analytics techniques and geospatial modeling to gain deeper insights into the interplay between human activities and land use patterns.
Traditional methods of analyzing geospatial data have relied on supervised learning approaches, where a model is trained using labeled data. However, these methods often face challenges when dealing with large-scale datasets or when new or unknown patterns emerge. The proposed self-supervised approach aims to overcome these limitations by leveraging the inherent structure of the data itself.
Unveiling the Underlying Themes and Concepts
By analyzing geospatial activity temporal patterns, it is possible to uncover hidden themes and concepts that underlie the relationship between human mobility and land use. For example, time-based patterns of activity can reveal peak hours of transportation usage, highlighting congested areas that may require targeted infrastructure improvements.
Moreover, understanding the temporal patterns can provide valuable insights into land use dynamics. Patterns such as increased activity in certain seasons or fluctuating trends over time can help identify areas that undergo transformations in land use, indicating potential opportunities for revitalization or conservation efforts.
Digitizing Mobility Patterns: The Power of Big Data
The advent of big data has revolutionized the field of geospatial analysis. With the ubiquity of GPS-enabled devices and the proliferation of mobile applications, vast amounts of data regarding human mobility are being generated every day. Leveraging this data and applying innovative algorithms, it is now possible to gain a granular understanding of geospatial activity patterns like never before.
“The ability to process and analyze large-scale mobility datasets provides an unprecedented opportunity to inform decision-making processes for urban planning, transportation management, and environmental sustainability.” – John Doe, geospatial analyst
Proposing Innovative Solutions and Ideas
The self-supervised approach opens up exciting possibilities for proposing innovative solutions and ideas in the realm of geospatial analysis. By understanding the relationship between human activity patterns and land use, decision-makers can develop targeted interventions to maximize efficiency, promote sustainable practices, and enhance the overall quality of life in urban environments.
For instance, identifying areas with high temporal variability in activity patterns can aid in designing flexible transportation systems that dynamically adapt to demand. Utilizing data on seasonal activity fluctuations can inform land use planning decisions, ensuring efficient resource allocation and facilitating adaptive urban development.
Conclusion
As we delve deeper into the world of geospatial analysis, the self-supervised approach proves to be a promising avenue for unraveling the correlation between geospatial activity temporal patterns and land use. By harnessing big data and leveraging advanced analytics techniques, researchers can generate valuable insights to drive informed decision-making and shape a more sustainable and livable future.
Geospatial activity refers to the movement and behavior of individuals within a given geographic area, typically captured through location-based services or GPS data. This data can provide valuable insights into various aspects of human behavior, including patterns of mobility and land use. Understanding the relationship between geospatial activity temporal patterns and land use can have significant implications for urban planning, transportation management, and resource allocation.
The proposed self-supervised approach to stratify landscape based on mobility activity time is an innovative and potentially powerful method for analyzing and categorizing land use patterns. Traditionally, land use classification has relied on manual surveys or remote sensing techniques, which can be time-consuming and limited in their ability to capture dynamic changes. By leveraging geospatial activity data, this approach offers a more granular and real-time understanding of how different areas are being utilized.
One key advantage of self-supervised learning is that it does not require labeled training data. Instead, it leverages the inherent structure and patterns within the geospatial activity data itself to identify meaningful clusters or categories. This is particularly useful in scenarios where ground truth labels may be unavailable or difficult to obtain.
By stratifying the landscape based on mobility activity time, this approach can potentially uncover hidden relationships between human behavior and land use. For example, it may reveal that certain areas experience high levels of pedestrian activity during specific times of the day, indicating the presence of commercial or retail areas. Similarly, areas with high vehicular movement during peak hours may be associated with industrial or transportation hubs.
Furthermore, this self-supervised approach can adapt and evolve as mobility patterns change over time. By continuously analyzing and updating the clustering or categorization of land use based on the latest geospatial activity data, planners and policymakers can make more informed decisions about zoning regulations, infrastructure development, and resource allocation.
However, it is important to note that this approach also comes with its own set of challenges and limitations. The quality and representativeness of the geospatial activity data can heavily influence the accuracy and reliability of the results. Issues such as data privacy, sampling biases, and data quality control need to be carefully addressed to ensure the validity of the findings.
In terms of future developments, there are several exciting directions this research could take. One potential avenue is to incorporate additional contextual information, such as socio-economic data, environmental factors, or demographic characteristics, to enhance the understanding of land use patterns. By integrating multiple data sources, researchers can gain a more holistic and nuanced perspective on the relationship between geospatial activity and land use.
Moreover, exploring the application of machine learning techniques, such as deep learning or reinforcement learning, could further improve the accuracy and predictive power of the self-supervised approach. These advanced algorithms can uncover complex patterns and dependencies within the geospatial activity data, enabling more accurate predictions of land use changes and better-informed decision-making.
Overall, the proposed self-supervised approach to stratify landscape based on mobility activity time holds great potential for advancing our understanding of land use patterns. By leveraging geospatial activity data, this approach can provide valuable insights into human behavior and help shape more efficient and sustainable urban environments. Continued research and innovation in this field will undoubtedly lead to new discoveries and practical applications in the realm of urban planning and management.
Read the original article