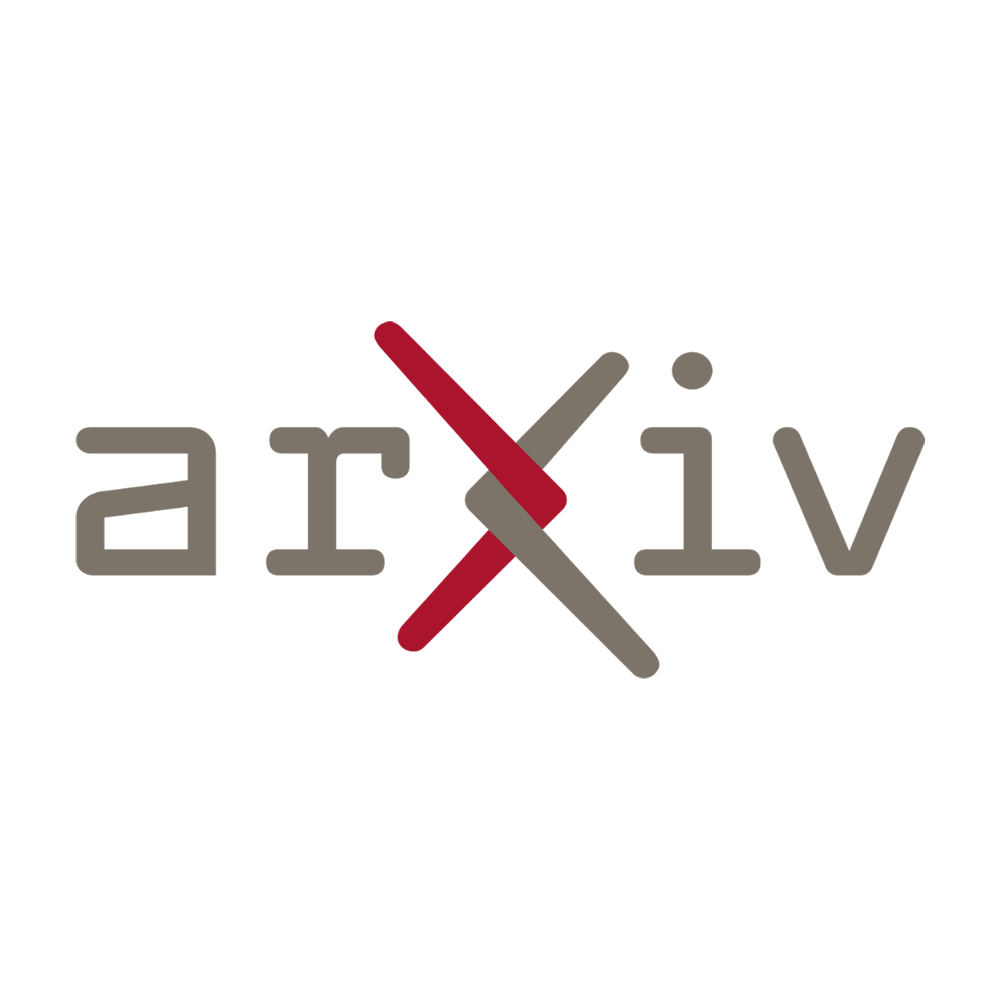
In the field of functional data analysis, a widely used approach involves transforming discrete data into smooth functions, followed by representing these functions using a finite-dimensional vector of coefficients. This process, known as the common pipeline in functional data analysis, allows researchers to extract valuable insights from complex data sets. By converting discrete observations into smooth functions, researchers can effectively capture the underlying patterns and trends in the data. These functions are then represented by a finite-dimensional vector of coefficients, which simplifies the data representation while retaining essential information. This article delves into the core themes of this common pipeline, highlighting its significance in functional data analysis and its potential to unlock new insights in various domains.
A common pipeline in functional data analysis is to first convert the discretely observed data to smooth functions, and then represent these functions by a finite-dimensional vector of coefficients. This approach allows us to analyze and interpret complex data sets using more traditional statistical techniques.
Exploring the Underlying Themes
At the heart of this approach lies the idea that functions can be represented as vectors in a high-dimensional space. This concept is particularly useful when dealing with data that naturally vary over a continuous domain, such as time or space. By representing functions using a finite number of coefficients, we can capture their essential characteristics while reducing the dimensionality of the data.
Functional data analysis opens up a world of possibilities for studying patterns and relationships in complex datasets. It allows us to apply well-established statistical methods, such as regression or clustering, to functional data. This, in turn, enables us to make predictions, uncover underlying structures, and gain insights from the data that might not be apparent at first glance.
Proposing Innovative Solutions and Ideas
While the techniques used in functional data analysis are powerful, there is always room for innovation and improvement. Here are some innovative solutions and ideas that can further enhance our understanding and analysis of functional data:
- Developing novel algorithms: As technology advances, we can develop new algorithms that handle larger and more complex datasets efficiently. These algorithms can leverage advances in machine learning and optimization techniques to extract even more information from functional data.
- Integrating functional and non-functional data: Combining functional data with other types of data, such as categorical or numerical variables, can provide a more comprehensive understanding of complex phenomena. Novel techniques that enable the integration of different types of data can open up new avenues for analysis and interpretation.
- Incorporating uncertainty: Many real-world datasets are subject to uncertainties and measurement errors. Developing methods that explicitly account for these uncertainties can improve the robustness and reliability of functional data analysis. Bayesian approaches or Monte Carlo simulations can be explored to incorporate uncertainty into the analysis.
- Beyond traditional statistical techniques: While traditional statistical methods have been successfully applied to functional data analysis, exploring alternative techniques can lead to new insights. Techniques from signal processing, graph theory, or time series analysis can be adapted and combined with the existing methodologies to uncover hidden patterns and relationships.
Conclusion
The conversion of discretely observed data into smooth functions, followed by their representation as finite-dimensional vectors, forms the foundation of functional data analysis. This approach allows us to analyze complex data sets by applying traditional statistical techniques within a functional framework. The exploration of underlying themes and the proposal of innovative solutions can further enhance our understanding and analysis of functional data, paving the way for new discoveries and insights.
“Functional data analysis opens up a world of possibilities for studying patterns and relationships in complex datasets.”
In functional data analysis, the goal is to analyze data that can be represented as smooth functions. However, many real-world datasets are observed discretely, meaning that we only have measurements at specific points in time or space. To overcome this limitation, a common approach is to convert the discrete data into smooth functions.
This conversion process involves fitting a curve or surface to the observed data points, effectively smoothing out any noise or irregularities. There are various techniques available for this purpose, such as spline interpolation, kernel smoothing, or Gaussian processes. Each method has its own strengths and weaknesses, depending on the characteristics of the data and the desired level of smoothness.
Once the data has been transformed into smooth functions, the next step is to represent these functions using a finite-dimensional vector of coefficients. This is done by selecting a suitable basis or set of basis functions that can effectively capture the variability and structure of the functions.
Commonly used basis functions include Fourier basis functions, B-spline basis functions, wavelet basis functions, or principal component basis functions. The choice of basis functions depends on the specific problem at hand and the nature of the functions being analyzed.
By representing the smooth functions with a finite-dimensional vector of coefficients, we can simplify the subsequent analysis and apply standard statistical techniques. This allows us to perform tasks such as hypothesis testing, regression analysis, or clustering on functional data.
Looking ahead, future developments in functional data analysis may focus on improving the accuracy and efficiency of the conversion from discrete data to smooth functions. This could involve advancements in computational algorithms or the development of new statistical models that can handle complex and high-dimensional functional data.
Additionally, there might be a growing emphasis on incorporating domain knowledge or prior information into the analysis. By leveraging expert insights and incorporating prior knowledge about the underlying processes generating the data, we can enhance the interpretability and predictive power of functional data analysis methods.
Overall, the pipeline of converting discretely observed data to smooth functions and representing them as a finite-dimensional vector of coefficients forms a fundamental framework in functional data analysis. It provides a powerful tool for extracting meaningful information from complex and continuous datasets, paving the way for further advancements in statistical modeling and inference in the field.
Read the original article