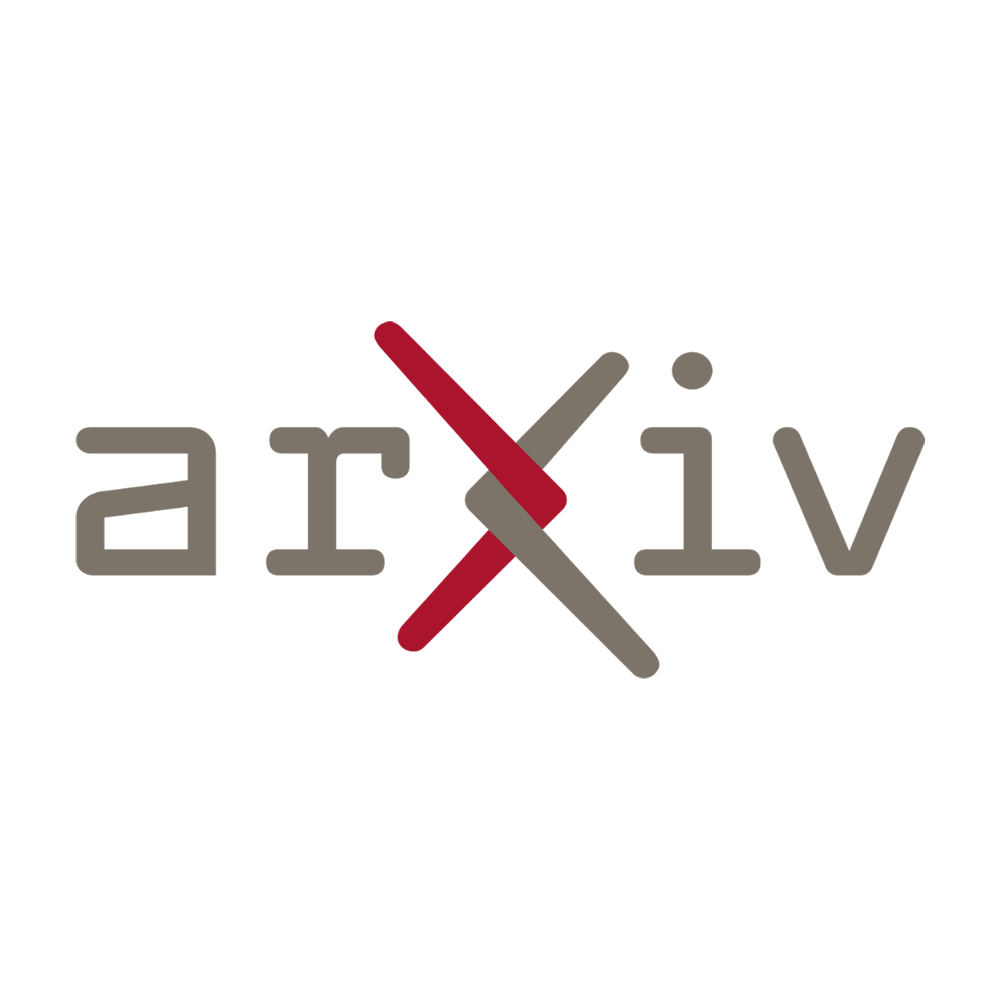
In today’s digital world, the evaluation of synthetic speech has become crucial in determining its quality and ensuring a seamless user experience. Automatic Mean Opinion Score (MOS) prediction has emerged as a valuable tool for this purpose, allowing us to assess the perceived quality of synthesized speech. However, the applications of predicted MOS extend beyond just speech evaluation. In a groundbreaking study, researchers have explored the use of predicted MOS in the task of Fake Audio Detection. This innovative approach aims to identify and differentiate between genuine and manipulated audio, shedding light on the alarming rise of fake audio content. By leveraging the power of predicted MOS, this study opens up new possibilities in combating audio manipulation and safeguarding the authenticity of audio content in our increasingly digital landscape.
Exploring the Link Between Predicted MOS and Fake Audio Detection
A new approach to identifying and combatting fake audio
The Importance of MOS Prediction
Automatic Mean Opinion Score (MOS) prediction has revolutionized the evaluation of synthetic speech, providing a quantitative measure of its quality. This technology, based on machine learning algorithms, allows us to assess speech synthesis systems quickly and accurately, saving time and resources.
Extending the Application of Predicted MOS
In a bold move, researchers have expanded the application of predicted MOS beyond speech quality evaluation. By leveraging the insights gained from MOS prediction, they have developed a new use case for this technology – detecting fake audio.
Fighting Audio Manipulation with Predicted MOS
The rise of deepfake technology has raised concerns about the authenticity and trustworthiness of audio content. With the ability to generate highly convincing fake audio, malicious actors can exploit this technology to spread misinformation or deceive unsuspecting individuals.
To combat this threat, researchers propose using predicted MOS as a tool for fake audio detection. By analyzing the perceptual quality features of both genuine and manipulated audio, machine learning models trained on predicted MOS data can learn to differentiate between real and fake audio content.
Challenges and Innovations in Fake Audio Detection
Applying MOS prediction to the task of fake audio detection presents several unique challenges. Unlike speech synthesis evaluation, where the goal is to assess the naturalness and intelligibility of synthetic speech, fake audio detection requires identifying subtle artifacts or anomalies that may indicate manipulation.
Researchers have developed innovative solutions to address these challenges. By focusing on specific perceptual quality features that tend to deviate in manipulated audio, such as spectral irregularities or inconsistencies in prosody, machine learning models can be trained to identify these patterns and classify audio as genuine or fake.
The Future of Fake Audio Detection
The integration of predicted MOS into the field of fake audio detection opens up exciting possibilities for combating audio manipulation. As machine learning algorithms improve and larger datasets of predicted MOS become available, the accuracy and reliability of fake audio detection systems will likely increase.
Furthermore, the use of predicted MOS adds an additional layer of credibility to these systems. By leveraging the established relationship between perceived quality and authenticity, predicted MOS can provide a robust metric for assessing the trustworthiness of audio content.
“With the advancement in predictive MOS technology, we now have a powerful tool to battle the rising tide of fake audio. By combining our knowledge of audio quality with machine learning, we can safeguard the integrity of audio content and protect individuals from deception.”
In conclusion
Predicted MOS, originally developed for speech synthesis evaluation, has emerged as a promising solution for identifying fake audio. By harnessing our understanding of perceptual quality features, machine learning models can be trained to detect subtle manipulation and distinguish genuine from fake audio content. With further research and advancements in this area, we can look forward to a future where audio content remains trustworthy and genuine.
Automatic Mean Opinion Score (MOS) prediction is a valuable tool in evaluating the quality of synthetic speech and plays a crucial role in applications such as text-to-speech systems. However, its application in the field of Fake Audio Detection is a novel and intriguing development that has the potential to greatly enhance the accuracy and efficiency of detecting manipulated or counterfeit audio content.
Fake audio, also known as audio deepfakes or synthetic speech forgery, has become a growing concern in recent years due to advancements in machine learning and speech synthesis technologies. These advancements have made it increasingly difficult to distinguish between genuine and artificially generated audio, leading to potential misuse and manipulation of audio content for malicious purposes such as spreading misinformation, impersonation, or fraud.
By extending the application of predicted MOS to the task of Fake Audio Detection, researchers aim to leverage the existing knowledge and techniques used in evaluating synthetic speech quality to identify and combat fake audio. This approach is based on the assumption that fake audio is likely to exhibit certain characteristics that can be detected by analyzing the quality of the synthesized speech.
One possible approach for utilizing predicted MOS in Fake Audio Detection involves training machine learning models using a dataset consisting of both genuine and fake audio samples. These models can be trained to learn patterns and features that are indicative of manipulated or synthetic audio. By extracting relevant acoustic features from the audio signals and using them as input to the models, it becomes possible to classify whether an audio sample is real or fake.
The integration of predicted MOS into this detection process can provide valuable insights into the quality and authenticity of the audio content. By analyzing the relationship between predicted MOS scores and the presence of fake audio, researchers can identify patterns and thresholds that can be used as indicators of potential manipulation. This analysis can help establish a baseline for distinguishing genuine from synthetic speech, allowing for more accurate detection of fake audio.
However, it’s important to note that while predicted MOS can be a powerful tool, it is not without limitations. The accuracy of MOS prediction depends on the quality and diversity of the training data used, as well as the robustness of the machine learning models employed. Additionally, the effectiveness of this approach may vary depending on the sophistication of the fake audio generation techniques being used.
Looking ahead, further research and development in this area are crucial for staying ahead of the evolving nature of fake audio. Continued efforts to improve the accuracy and efficiency of MOS prediction models, as well as the exploration of additional features and techniques, will be essential in tackling the challenges posed by fake audio. Furthermore, collaboration between researchers, industry experts, and policymakers will be vital in developing effective countermeasures and regulations to mitigate the risks associated with fake audio.
Read the original article