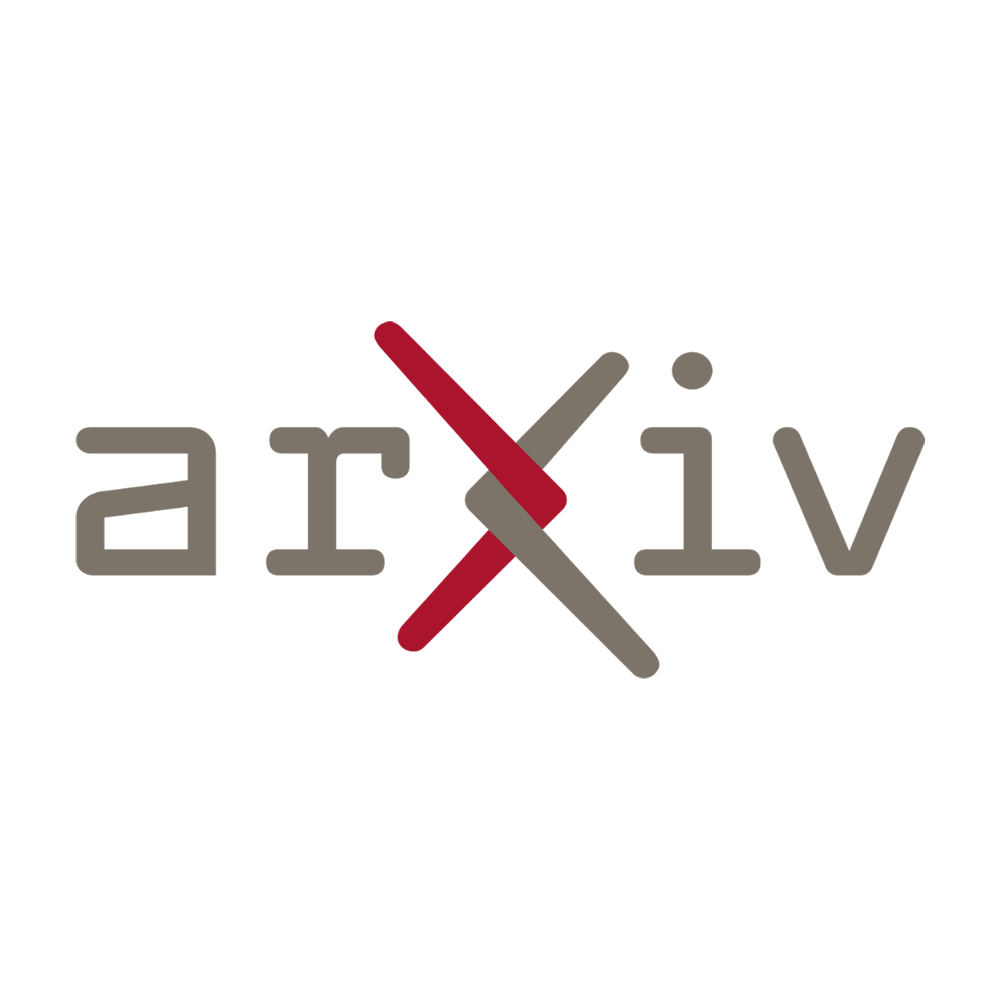
the complex decision-making processes and cognitive biases that influence their behavior. In this article, we delve into the intricacies of modeling subrational agents, such as humans or economic households, and explore the challenges that arise when trying to calibrate reinforcement learning models or collect data that accurately captures their decision-making processes. By understanding these challenges, we gain valuable insights into the limitations of current modeling techniques and the potential implications for various fields, including economics, psychology, and artificial intelligence. Join us as we navigate the complexities of modeling subrational agents and uncover the key factors that shape their behavior.
Modeling subrational agents, such as humans or economic households, is inherently challenging due to the difficulty in calibrating reinforcement learning models or collecting data that involves complex human decision-making processes. However, recent advancements in machine learning and simulation techniques are offering innovative solutions for understanding and predicting the behavior of such agents.
The Complexity of Modeling Subrational Agents
Subrational agents, which can include humans, animals, or economic entities, exhibit decision-making that is driven by a combination of emotions, biases, and cognitive limitations. This makes it challenging to create accurate models that can capture the intricacies of their behavior. Traditional approaches often rely on rational choice theory, which assumes individuals make decisions based on the maximization of their utility. However, this framework falls short in explaining real-world behavior.
Reinforcement learning models have gained popularity in capturing subrational behavior by focusing on how agents learn from experience and their interactions with the environment. However, these models require extensive calibration, which can be difficult when dealing with complex human decision-making processes. Additionally, data collection for such models can be limited and biased.
Innovative Solutions: Advancements in Machine Learning
Advancements in machine learning techniques have presented promising solutions for modeling subrational agents. One approach involves using deep reinforcement learning algorithms that combine the power of neural networks with reinforcement learning principles. These models have the potential to capture more nuanced behavior by learning from large amounts of simulated or real-world data.
Simulated environments offer a controlled setting for studying subrational behavior. By creating virtual worlds or economic simulations, researchers can collect vast amounts of data on agent interactions and decision-making processes. This enables the calibration of reinforcement learning models without the limitations of collecting real-world data.
Another innovative solution is the use of generative adversarial networks (GANs) to generate simulated data. GANs can create realistic synthetic data that mimic the behavior of subrational agents. These synthetic datasets can then be used to train reinforcement learning models, capturing the complexities of human decision-making without relying solely on limited real-world data.
The Implications of Understanding Subrational Behavior
Understanding and modeling subrational behavior have diverse implications across various fields. In economics, accurate models of household decision-making could aid in policy design and create interventions that align with human behavior. In psychology, these models can enhance our understanding of cognitive biases and emotional decision-making.
This understanding can also be valuable in designing artificial intelligence systems that interact with humans. By modeling and simulating subrational behavior, researchers can create AI algorithms that are more empathetic and responsive to human needs and emotions. This could lead to advancements in customer service, healthcare, and other domains where human interaction is crucial.
In summary, while modeling subrational agents poses inherent challenges, advancements in machine learning and simulation techniques offer innovative solutions for capturing and understanding their behavior. These approaches enable researchers to study complex decision-making processes, leading to a better understanding of human behavior and the development of applications across various domains.
complex human decision-making processes. While reinforcement learning models have shown great success in modeling rational agents in controlled environments, applying them to subrational agents introduces several challenges.
One key challenge is calibrating reinforcement learning models to capture the intricacies of human decision-making. Unlike rational agents that optimize their actions based on a well-defined utility function, humans often exhibit biases, heuristics, and subjective preferences that are difficult to quantify. These complexities make it challenging to design an accurate model that captures the nuances of human behavior.
Furthermore, collecting data that accurately represents the decision-making processes of subrational agents is a daunting task. Humans and economic households make decisions based on a wide range of factors, including emotions, social context, and long-term goals. Gathering comprehensive and representative data that encompasses these variables is a complex endeavor. Moreover, the collection process itself may introduce biases and limitations, further complicating the modeling process.
To tackle these challenges, researchers have started exploring alternative approaches. One promising direction is the development of hybrid models that combine reinforcement learning with other techniques, such as cognitive psychology or behavioral economics. By integrating insights from these disciplines, we can improve the fidelity of models and better capture the complexities of subrational decision-making.
Another avenue of research involves using observational data rather than relying solely on controlled experiments. Observational data provides a more realistic glimpse into how humans and economic households make decisions in their natural environments. However, leveraging observational data poses its own set of challenges, such as dealing with confounding factors and ensuring the data represents a diverse range of decision-making scenarios.
In the future, advancements in technology and data collection methods may help address some of these challenges. For instance, advancements in wearable devices and ubiquitous sensing could provide more fine-grained data on human behavior and decision-making processes. Additionally, advancements in machine learning techniques, such as transfer learning or meta-learning, may offer ways to leverage existing models trained on related tasks or domains to bootstrap the modeling of subrational agents.
Overall, modeling subrational agents is a complex and evolving field. While significant challenges remain in calibrating reinforcement learning models and collecting relevant data, researchers are actively exploring innovative approaches to improve the fidelity of these models. By combining insights from psychology, economics, and machine learning, we can hope to develop more accurate and comprehensive models that better capture the intricacies of human decision-making.
Read the original article