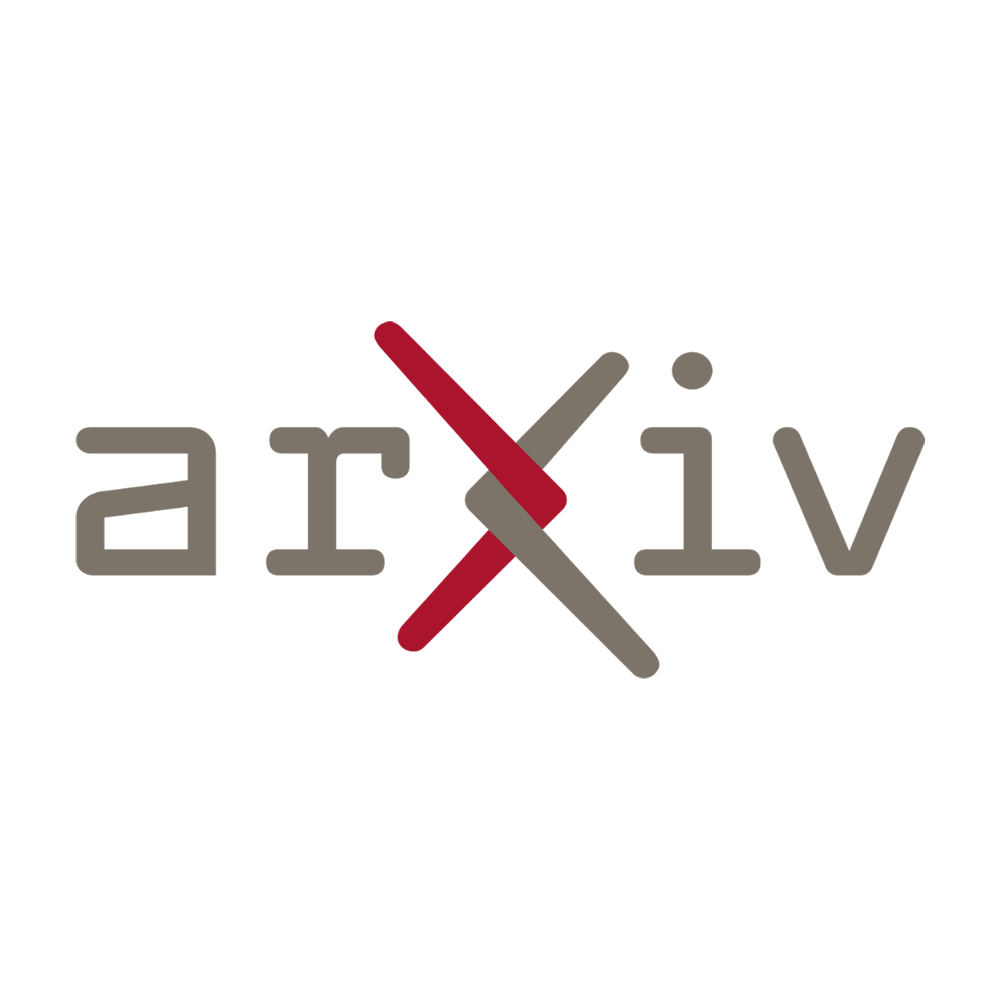
In today’s digital age, where user-generated content is abundant, identifying helpful reviews has become a challenging task. Researchers have recognized the importance of distinguishing valuable information from the vast pool of textual and visual data. This article delves into the latest research and strategies employed to effectively identify helpful reviews. By leveraging various modalities and employing advanced techniques, researchers aim to provide users with the most relevant and informative reviews, enhancing their decision-making process.
In line with the latest research, the task of identifying helpful reviews from a vast pool of user-generated textual and visual data has become a prominent area of study. Effective modalities to help users quickly identify useful and relevant information are crucial in today’s digital landscape. In this article, we will explore the underlying themes and concepts related to helpful reviews, proposing innovative solutions and ideas to enhance the experience of both reviewers and users.
The Importance of Helpful Reviews
Helpful reviews serve as a guiding light for consumers, assisting them in making informed purchasing decisions. They offer insights, experiences, and opinions from previous customers, helping potential buyers assess product quality, features, and suitability to their needs. However, the sheer volume of user-generated content can make finding helpful reviews a daunting task.
Distinguishing between Helpful and Unhelpful Reviews
One of the main challenges lies in distinguishing between helpful and unhelpful reviews. While some reviews offer detailed analyses and practical information, others may consist of generic statements or biased opinions. To address this issue, leveraging natural language processing (NLP) techniques can prove highly effective.
Tip: Using sentiment analysis, a subfield of NLP, can help identify the sentiment expressed in reviews. This can be a useful indicator for potential helpfulness, as positive sentiment reviews are often seen as more trustworthy and relevant.
Visual Elements Enhancing Review Engagement
Another aspect to consider is the inclusion of visual elements in reviews, such as images or videos. These elements can significantly enhance review engagement and make information more easily digestible. For example, a user searching for a hotel review will likely find images of the room layout, amenities, or views much more valuable when making their decision.
Idea: Implementing a review platform that encourages users to upload relevant images or short videos alongside their text reviews can provide a comprehensive and immersive experience for potential buyers.
Personalization and Recommender Systems
Personalization is becoming increasingly crucial in the digital realm. Recommender systems can play a vital role in helping users find relevant and helpful reviews by tailoring recommendations based on their preferences, past reviews, or browsing history. This approach not only saves time for the user but also ensures they receive information that aligns with their specific needs and interests.
Idea: A personalized review platform that utilizes recommender systems can significantly improve the user experience, increase engagement, and promote trust in the reviews provided.
Building a Community and Promoting Collaboration
Creating a sense of community and promoting collaboration among reviewers can foster a more interactive and informative review environment. Allowing users to interact with each other, ask questions, and provide feedback not only enhances the credibility of reviews but also encourages knowledge-sharing and a sense of collective responsibility.
Idea: Implementing a comment section or a discussion forum within the review platform can facilitate engagement, promote collaboration, and enable users to seek clarification or further details on specific aspects.
The Future of Helpful Reviews
The field of reviewing and accessing helpful user-generated content is continuously evolving. New technologies like machine learning, artificial intelligence, and augmented reality hold immense potential in revolutionizing how we perceive and utilize reviews.
As technology advances, leveraging these tools to develop intelligent systems that automatically curate, summarize, and prioritize helpful reviews will become increasingly important. Additionally, integrating user feedback mechanisms, such as user ratings for review helpfulness, can further enhance the assessment process.
Idea: A future vision could involve interactive augmented reality platforms where users can virtually experience products and read contextually relevant reviews, providing a more immersive and informed decision-making experience.
Conclusion
Identifying helpful reviews from the vast amount of user-generated content is a complex challenge. However, by leveraging innovative approaches such as sentiment analysis, visual elements, personalized recommendations, community-building features, and emerging technologies, we can enhance the review experience for users and ensure they receive the information they need to make informed decisions. The future holds exciting possibilities for the evolution of helpful reviews, and through continuous research and technological advancements, we can create a more user-centric and knowledge-driven review ecosystem.
Effective modalities for review identification are crucial for both businesses and consumers in today’s digital landscape. With the exponential growth of user-generated content, it has become increasingly challenging to sift through the vast amount of textual and visual data to identify helpful reviews. However, recent research has made significant progress in this area, paving the way for exciting developments and potential applications.
One promising approach to review identification is the use of natural language processing (NLP) techniques. NLP allows for the analysis of textual data to extract meaningful insights and sentiment. By leveraging NLP algorithms, researchers have been able to develop models that can automatically identify helpful reviews based on various criteria such as relevance, quality, and usefulness. These models can sift through large volumes of user-generated content and provide valuable recommendations to businesses and consumers alike.
Visual data, such as images and videos, also play a crucial role in the review identification process. In an era where visual content is increasingly prevalent, it is essential to develop methods that can effectively analyze and interpret these types of data. Computer vision techniques, combined with machine learning algorithms, have shown promising results in extracting relevant information from visual reviews. These methods can analyze images or videos associated with a review, identifying key features or patterns that contribute to its helpfulness.
Furthermore, incorporating user preferences and personalized recommendations into the review identification process can enhance the overall accuracy and usefulness of the identified reviews. By leveraging user-specific data, such as past preferences, purchase history, or browsing behavior, personalized models can tailor the review identification process to individual users’ needs and preferences. This approach can help businesses provide more targeted recommendations and allow consumers to find reviews that align with their specific interests and requirements.
Looking ahead, the future of review identification lies in the integration of multiple modalities, combining textual, visual, and even audio data. By leveraging the strengths of each modality and developing sophisticated multi-modal models, researchers can unlock deeper insights and improve the accuracy of review identification. For example, analyzing the sentiment expressed in an image or video alongside the accompanying textual review can provide a more comprehensive understanding of its helpfulness.
Additionally, advancements in deep learning techniques, such as deep neural networks and transformers, hold great promise for the field of review identification. These models have shown exceptional performance in various natural language processing and computer vision tasks, and their application to review identification can potentially revolutionize the field. Deep learning models can capture complex patterns and dependencies within textual and visual data, enabling more accurate and robust identification of helpful reviews.
In conclusion, the task of identifying helpful reviews from a vast pool of user-generated textual and visual data is an active area of study. Recent research has made significant strides in developing effective modalities for review identification, leveraging natural language processing, computer vision, and personalized recommendations. The integration of multiple modalities and the application of advanced deep learning techniques hold great promise for the future, enabling more accurate and comprehensive identification of helpful reviews. These advancements will benefit businesses in making informed decisions and consumers in finding trustworthy and relevant information.
Read the original article