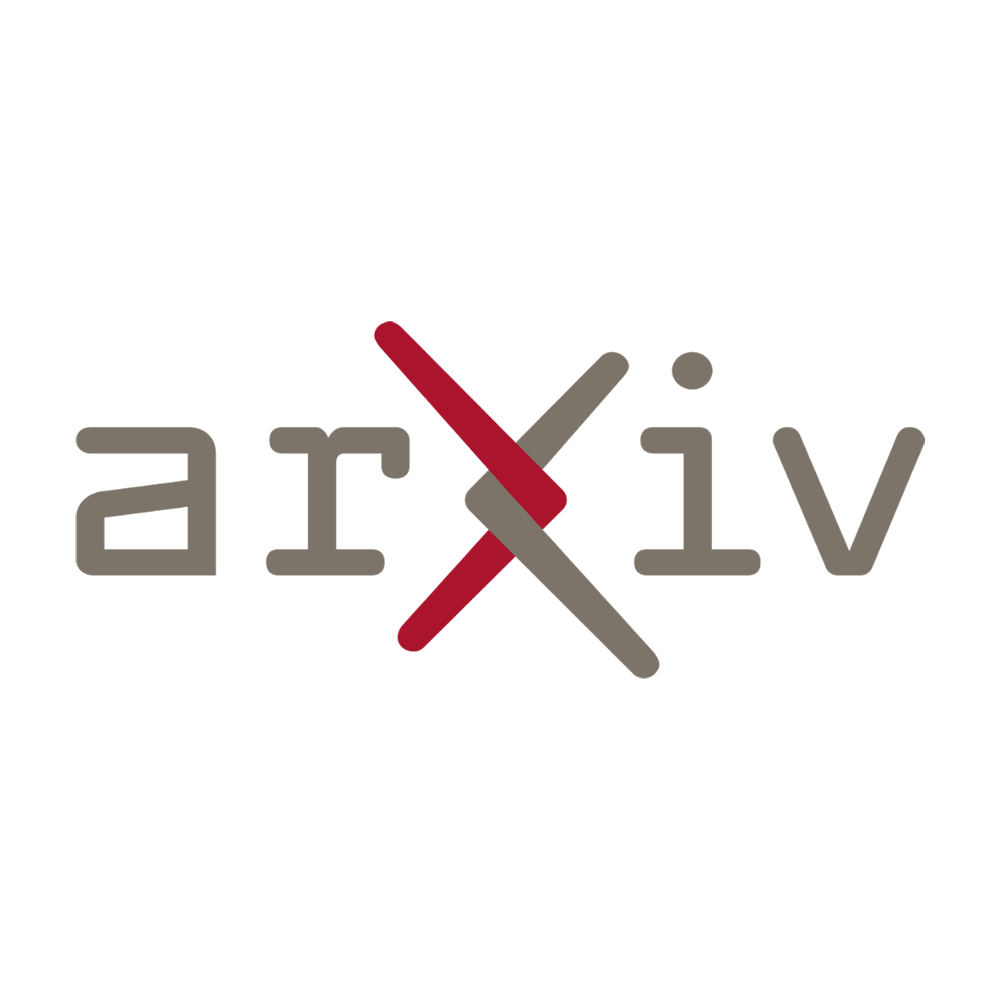
address the challenges of domain adaptation in a more comprehensive manner. SF-UniDA focuses on knowledge transfer when faced with both changes in the input data distribution (covariate shift) and changes in the output label distribution (label shift). This article explores the concept of SF-UniDA and its potential to overcome the limitations of traditional domain adaptation methods. By eliminating the need for source domain data, SF-UniDA offers a more flexible and practical approach to domain adaptation, making it a promising solution for real-world applications.
Universal Domain Adaptation (UniDA) is a powerful technique that focuses on transferring knowledge in situations where there are shifts in both the covariate (input features) and the label (output classes) spaces. This field has seen significant developments in recent years, particularly with the emergence of Source-free Universal Domain Adaptation (SF-UniDA).
SF-UniDA takes UniDA one step further by eliminating the need for a source domain, meaning that it can adapt to a new target domain without requiring any prior knowledge from a source domain. This breakthrough opens up new possibilities and challenges the traditional paradigm of domain adaptation.
The underlying concept of SF-UniDA
The underlying concept of SF-UniDA is to exploit the relationships between the target domain and a reference dataset, usually a large-scale public dataset, to learn a domain-invariant representation. By leveraging the information contained in the reference dataset, SF-UniDA can overcome the lack of labeled examples in the target domain, making it a highly practical and versatile approach.
This raises an interesting question: can SF-UniDA achieve similar or even better performance compared to traditional UniDA methods that rely on a source domain? The answer lies in the inherent flexibility and adaptability of SF-UniDA. By learning from the reference dataset and adjusting the model accordingly, SF-UniDA can effectively handle a wide range of domain shifts, even those that are not directly related to the source domain.
Innovative solutions offered by SF-UniDA
The introduction of SF-UniDA brings forth a set of innovative solutions to long-standing challenges in domain adaptation. One of the key advantages is the ability to adapt to novel target domains without any prior source knowledge. This allows for seamless integration of new data sources in various domains, making SF-UniDA a valuable tool for industries with rapidly changing environments.
Furthermore, SF-UniDA’s reliance on a reference dataset rather than a source domain opens up opportunities for cross-domain knowledge transfer. The reference dataset acts as a bridge between different domains, enabling the transfer of information that may not be directly available in the target domain. This cross-domain knowledge transfer can greatly enhance the adaptability and generalization capabilities of SF-UniDA.
Proposing new ideas for SF-UniDA
While SF-UniDA has already made significant strides, there are still avenues for further innovation and improvement. One possibility is the exploration of ensemble techniques for SF-UniDA, where multiple reference datasets are used to create a more robust and diverse training framework. By combining the strengths of different reference datasets, SF-UniDA could achieve even better performance across a wide range of target domains.
Another interesting direction is the integration of active learning techniques with SF-UniDA. Traditional domain adaptation methods often struggle with limited labeled data in the target domain. By incorporating active learning, SF-UniDA could actively select the most informative examples to label, improving its performance while requiring fewer labeled samples.
Overall, SF-UniDA represents a groundbreaking advancement in the field of domain adaptation. Its ability to adapt to novel target domains without any prior source knowledge, coupled with the potential for cross-domain knowledge transfer, opens up exciting possibilities for a wide range of applications. As researchers and practitioners continue to explore and innovate with SF-UniDA, we can expect further advancements and breakthroughs in the field of domain adaptation.
address the limitations of traditional domain adaptation methods. SF-UniDA aims to overcome the need for labeled source domain data, which can be expensive and time-consuming to obtain. This is a significant advancement in the field as it allows for more flexible and scalable domain adaptation techniques.
One of the key challenges in domain adaptation is dealing with both covariate and label shifts. Covariate shift refers to a difference in the distribution of input features between the source and target domains, while label shift occurs when the conditional distribution of labels given the input features changes across domains. Universal Domain Adaptation (UniDA) approaches tackle both these shifts to ensure effective knowledge transfer.
SF-UniDA takes this a step further by eliminating the reliance on labeled source domain data. Instead, it leverages the unlabeled target domain data to learn a domain-invariant representation. This approach is particularly useful in scenarios where obtaining labeled source data is difficult or impossible, such as in some real-world applications or when the source domain is not available.
By not requiring source domain labels, SF-UniDA opens up possibilities for broader application of domain adaptation techniques. It reduces the burden of data collection and annotation, making it more feasible to adapt models to new domains or continuously update them with evolving target data. This can be especially valuable in dynamic environments where the target domain may change over time.
The success of SF-UniDA relies on effective domain-invariant representation learning. It involves finding a latent space where the distributions of source and target domains align, despite their differences in covariate and label distributions. Various methods, such as adversarial learning and self-training, have been explored in this context to encourage domain-invariant feature extraction.
Looking ahead, the field of Universal Domain Adaptation is likely to witness further advancements. Researchers will continue to explore novel techniques for domain-invariant representation learning and address the challenges of more complex domain shifts. Additionally, efforts may be made to combine SF-UniDA with other domain adaptation approaches to further improve performance and adaptability.
One potential direction for future research is to investigate how SF-UniDA can be extended to handle partial label information in the source domain. In many real-world scenarios, it is common to have some labeled data from the source domain, even if it is limited. Incorporating this partial label information could potentially enhance the adaptation performance.
Overall, Source-free Universal Domain Adaptation (SF-UniDA) is a promising development in the field of domain adaptation. By eliminating the need for labeled source domain data, it opens up new possibilities for scalable and flexible knowledge transfer across domains. Continued research and innovation in this area hold the potential to enable robust and efficient adaptation to diverse real-world scenarios.
Read the original article