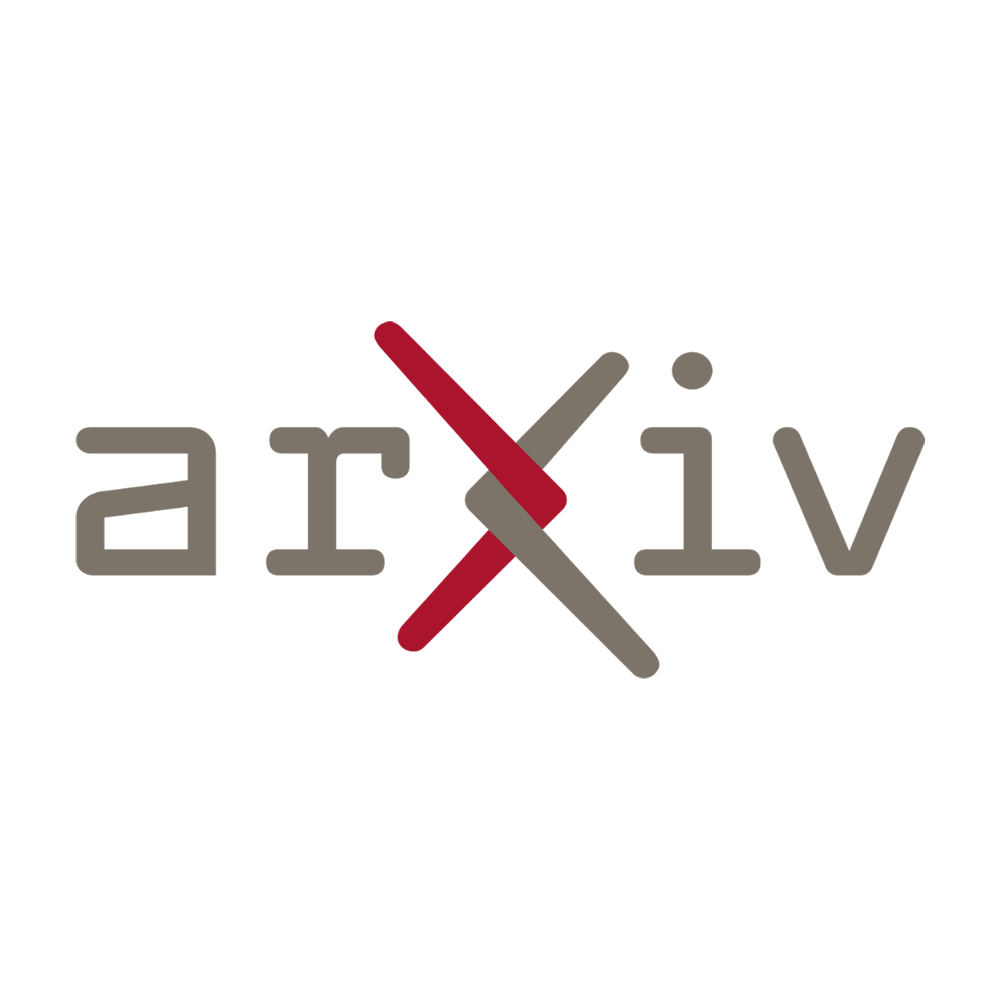
to the complex nature of music and the lack of standardized evaluation metrics, developing such benchmarks has proven to be a challenging task. In this article, we delve into the pressing need for new benchmarks to assess the capabilities of multimodal LLMs in understanding and describing music. As these models continue to advance at an unprecedented pace, it becomes crucial to have standardized measures that can comprehensively evaluate their performance. We explore the obstacles faced in creating these benchmarks and discuss potential solutions that can drive the development of improved evaluation metrics. By addressing this critical issue, we aim to pave the way for advancements in multimodal LLMs and their application in the realm of music understanding and description.
Proposing New Benchmarks for Evaluating Multimodal Large Language Models
The rapidly evolving multimodal Large Language Models (LLMs) urgently require new benchmarks to uniformly evaluate their performance on understanding and textually describing music. However, due to the complexity and subjective nature of musical comprehension, traditional evaluation methods often fall short in providing consistent and accurate assessments.
Music is a multifaceted art form that encompasses various structured patterns, emotional expressions, and unique interpretations. Evaluating an LLM’s understanding and description of music should consider these elements holistically. Instead of relying solely on quantitative metrics, a more comprehensive evaluation approach is needed to gauge the model’s ability to comprehend and convey the essence of music through text.
Multimodal Evaluation Benchmarks
To address the current evaluation gap, it is essential to design new benchmarks that combine both quantitative and qualitative measures. These benchmarks can be categorized into three main areas:
- Appreciation of Musical Structure: LLMs should be evaluated on their understanding of various musical components such as melody, rhythm, harmony, and form. Assessing their ability to describe these elements accurately and with contextual knowledge would provide valuable insights into the model’s comprehension capabilities.
- Emotional Representation: Music evokes emotions, and a successful LLM should be able to capture and describe the emotions conveyed by a piece of music effectively. Developing benchmarks that evaluate the model’s emotional comprehension and its ability to articulate these emotions in descriptive text can provide a deeper understanding of its capabilities.
- Creative Interpretation: Music interpretation is subjective, and different listeners may have unique perspectives on a musical piece. Evaluating an LLM’s capacity to generate diverse and creative descriptions that encompass various interpretations of a given piece can offer insights into its flexibility and intelligence.
By combining these benchmarks, a more holistic evaluation of multimodal LLMs can be achieved. It is crucial to involve experts from the fields of musicology, linguistics, and artificial intelligence to develop these benchmarks collaboratively, ensuring the assessments are comprehensive and accurate.
Importance of User Feedback
While benchmarks provide objective evaluation measures, it is equally important to gather user feedback and subjective opinions to assess the effectiveness and usability of multimodal LLMs in real-world applications. User studies, surveys, and focus groups can provide valuable insights into how well these models meet the needs and expectations of their intended audience.
“To unlock the full potential of multimodal LLMs, we must develop benchmarks that go beyond quantitative metrics and account for the nuanced understanding of music. Incorporating subjective evaluations and user feedback is key to ensuring these models have practical applications in enhancing music experiences.”
As the development of multimodal LLMs progresses, ongoing refinement and updating of the evaluation benchmarks will be necessary to keep up with the evolving capabilities of these models. Continued collaboration between researchers, practitioners, and music enthusiasts is pivotal in establishing a standard framework that can guide the development, evaluation, and application of multimodal LLMs in the music domain.
to the complex and subjective nature of music, creating a comprehensive benchmark for evaluating LLMs’ understanding and description of music poses a significant challenge. Music is a multifaceted art form that encompasses various elements such as melody, rhythm, harmony, lyrics, and emotional expression, making it inherently difficult to quantify and evaluate.
One of the primary obstacles in benchmarking LLMs for music understanding is the lack of a standardized dataset that covers a wide range of musical genres, styles, and cultural contexts. Existing datasets often focus on specific genres or limited musical aspects, which hinders the development of a holistic evaluation framework. To address this, researchers and experts in the field need to collaborate and curate a diverse and inclusive dataset that represents the vast musical landscape.
Another critical aspect to consider is the evaluation metrics for LLMs’ music understanding. Traditional metrics like accuracy or perplexity may not be sufficient to capture the nuanced nature of music. Music comprehension involves not only understanding the lyrics but also interpreting the emotional context, capturing the stylistic elements, and recognizing cultural references. Developing novel evaluation metrics that encompass these aspects is crucial to accurately assess LLMs’ performance in music understanding.
Furthermore, LLMs’ ability to textually describe music requires a deeper understanding of the underlying musical structure and aesthetics. While LLMs have shown promising results in generating descriptive text, there is still room for improvement. Future benchmarks should focus on evaluating LLMs’ capacity to generate coherent and contextually relevant descriptions that capture the essence of different musical genres and evoke the intended emotions.
To overcome these challenges, interdisciplinary collaborations between experts in natural language processing, music theory, and cognitive psychology are essential. By combining their expertise, researchers can develop comprehensive benchmarks that not only evaluate LLMs’ performance but also shed light on the limitations and areas for improvement.
Looking ahead, advancements in multimodal learning techniques, such as incorporating audio and visual information alongside textual data, hold great potential for enhancing LLMs’ understanding and description of music. Integrating these modalities can provide a more holistic representation of music and enable LLMs to capture the intricate interplay between lyrics, melody, rhythm, and emotions. Consequently, future benchmarks should consider incorporating multimodal data to evaluate LLMs’ performance comprehensively.
In summary, the rapidly evolving multimodal LLMs require new benchmarks to evaluate their understanding and textual description of music. Overcoming the challenges posed by the complex and subjective nature of music, the lack of standardized datasets, and the need for novel evaluation metrics will be crucial. Interdisciplinary collaborations and the integration of multimodal learning techniques hold the key to advancing LLMs’ capabilities in music understanding and description. By addressing these issues, we can pave the way for LLMs to become powerful tools for analyzing and describing music in diverse contexts.
Read the original article