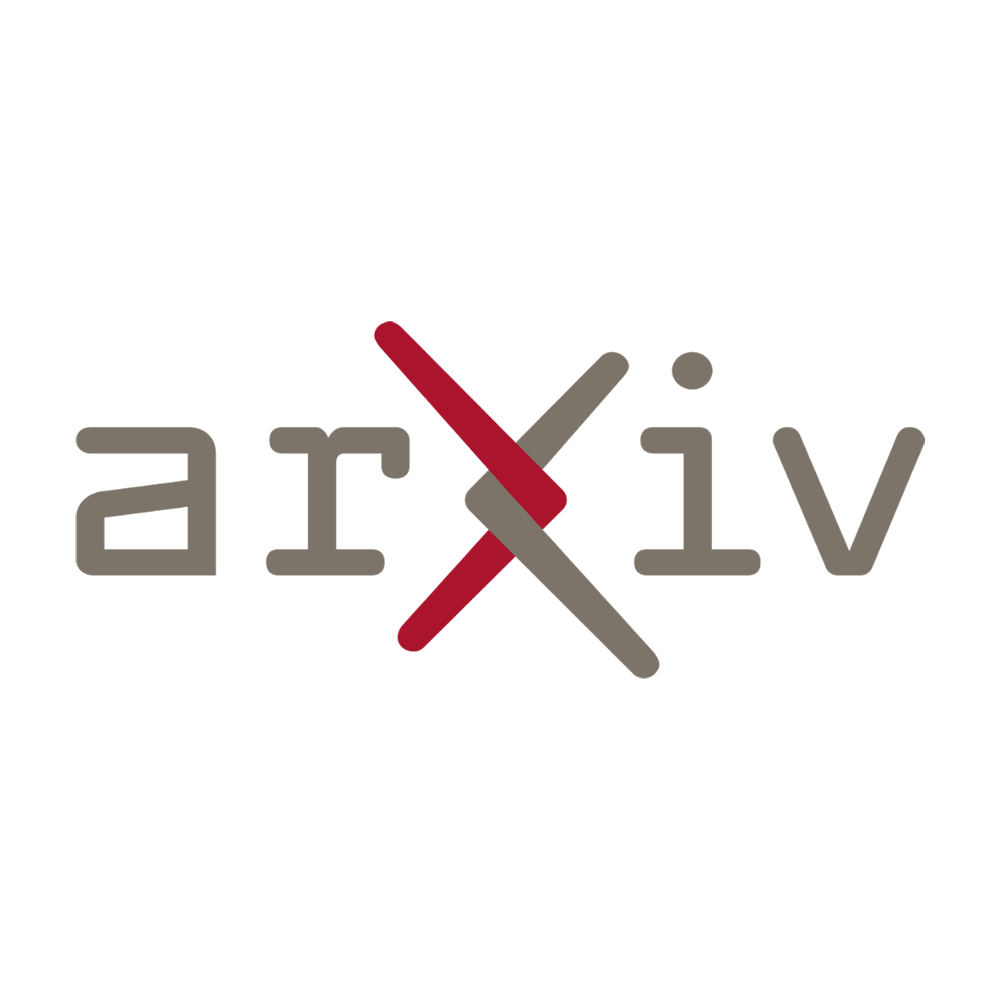
In the world of machine learning, the availability of labeled data has always been a key factor in advancing the field. However, the traditional methods of obtaining labeled data have proven to be time-consuming and costly. But now, thanks to the revolutionary concept of crowdsourcing annotations, a paradigm shift has occurred, opening up a whole new world of possibilities for machine learning researchers. This article explores how crowdsourcing annotations has transformed the availability of labeled data and accelerated progress in common knowledge. By harnessing the power of the crowd, machine learning practitioners can now access large datasets that were previously unimaginable, leading to significant advancements in various domains. Let’s delve into this groundbreaking approach and discover how it is reshaping the landscape of machine learning.
Crowdsourcing annotations has created a paradigm shift in the availability of labeled data for machine learning. Availability of large datasets has accelerated progress in common knowledge, but what about rare or niche topics? How can we ensure that machine learning models have access to specific and specialized information?
The Limitations of Crowdsourcing Annotations
Crowdsourcing annotations have revolutionized the field of machine learning by providing vast amounts of labeled data. By outsourcing the task to a large group of individuals, it becomes possible to annotate large datasets quickly and efficiently. However, there are inherent limitations to this approach.
One major limitation is the availability of expertise. Crowdsourced annotation platforms often rely on the general public to label data, which may not have the necessary domain knowledge or expertise to accurately label specific types of data. This becomes especially problematic when dealing with rare or niche topics that require specialized knowledge.
Another limitation is the lack of consistency in annotation quality. Crowdsourcing platforms often consist of contributors with varying levels of expertise and commitment. This can lead to inconsistencies in labeling, impacting the overall quality and reliability of the annotated data. Without a standardized process for verification and quality control, it is challenging to ensure the accuracy and integrity of the labeled data.
Introducing Expert Crowdsourcing
To address these limitations, we propose the concept of “Expert Crowdsourcing.” Rather than relying solely on the general public, this approach leverages the collective knowledge and expertise of domain-specific experts.
The first step is to create a curated pool of experts in the relevant field. These experts can be sourced from academic institutions, industry professionals, or even verified users on specialized platforms. By tapping into the existing knowledge of experts, we can ensure accurate and reliable annotations.
Once the pool of experts is established, a standardized verification process can be implemented. This process would involve assessing the expertise and reliability of each expert, ensuring that they are qualified to annotate the specific type of data. By maintaining a high standard of expertise, we can ensure consistency and accuracy in the annotations.
The Benefits of Expert Crowdsourcing
Implementing expert crowdsourcing can greatly improve the overall quality and availability of labeled data for machine learning models. By leveraging the knowledge of domain-specific experts, models can access specialized information that would otherwise be challenging to obtain.
Improved accuracy is another significant benefit. With experts annotating the data, the chances of mislabeling or inconsistent annotations are greatly reduced. Models trained on high-quality, expert-annotated data are likely to exhibit better performance and reliability.
Furthermore, expert crowdsourcing allows for the possibility of fine-grained annotations. Experts can provide nuanced and detailed labels that capture the intricacies of the data, enabling machine learning models to learn more sophisticated patterns and make more informed decisions.
Conclusion
Crowdsourcing annotations have undoubtedly revolutionized the field of machine learning. However, it is imperative to recognize the limitations of traditional crowdsourcing and explore alternative approaches such as expert crowdsourcing. By leveraging the knowledge and expertise of domain-specific experts, we can overcome the challenges of annotating rare or niche topics and achieve even greater progress in machine learning applications.
and natural language processing tasks. Crowdsourcing annotations involves outsourcing the task of labeling data to a large number of individuals, typically through online platforms, allowing for the rapid collection of labeled data at a much larger scale than traditional methods.
This paradigm shift has had a profound impact on the field of machine learning. Previously, the scarcity of labeled data posed a significant challenge to researchers and developers. Creating labeled datasets required substantial time, effort, and resources, often limiting the scope and applicability of machine learning models. However, with the advent of crowdsourcing annotations, the availability of large datasets has revolutionized the field by enabling more robust and accurate models.
One of the key advantages of crowdsourcing annotations is the ability to tap into a diverse pool of annotators. This diversity helps in mitigating biases and improving the overall quality of the labeled data. By distributing the annotation task among numerous individuals, the reliance on a single expert’s judgment is reduced, leading to more comprehensive and reliable annotations.
Moreover, the scalability of crowdsourcing annotations allows for the collection of data on a massive scale. This is particularly beneficial for tasks that require a vast amount of labeled data, such as image recognition or sentiment analysis. The ability to quickly gather a large number of annotations significantly accelerates the training process of machine learning models, leading to faster and more accurate results.
However, crowdsourcing annotations also present several challenges that need to be addressed. One major concern is the quality control of annotations. With a large number of annotators, ensuring consistent and accurate labeling becomes crucial. Developing robust mechanisms to verify the quality of annotations, such as using gold standard data or implementing quality control checks, is essential to maintain the integrity of the labeled datasets.
Another challenge is the potential for biases in annotations. As annotators come from diverse backgrounds and perspectives, biases can inadvertently be introduced into the labeled data. Addressing this issue requires careful selection of annotators and implementing mechanisms to detect and mitigate biases during the annotation process.
Looking ahead, the future of crowdsourcing annotations in machine learning holds great promise. As technology continues to advance, we can expect more sophisticated platforms that enable better collaboration, communication, and feedback between annotators and researchers. Additionally, advancements in artificial intelligence, particularly in the area of automated annotation and active learning, may further enhance the efficiency and accuracy of crowdsourcing annotations.
Furthermore, the integration of crowdsourcing annotations with other emerging technologies, such as blockchain, could potentially address the challenges of quality control and bias detection. Blockchain-based platforms can provide transparency and traceability, ensuring that annotations are reliable and free from manipulation.
In conclusion, crowdsourcing annotations have revolutionized the availability of labeled data for machine learning, fostering progress in common knowledge and natural language processing tasks. While challenges related to quality control and biases persist, the future holds great potential for further advancements in this field. By leveraging the power of crowdsourcing annotations and integrating it with evolving technologies, we can expect even greater breakthroughs in the development of robust and accurate machine learning models.
Read the original article