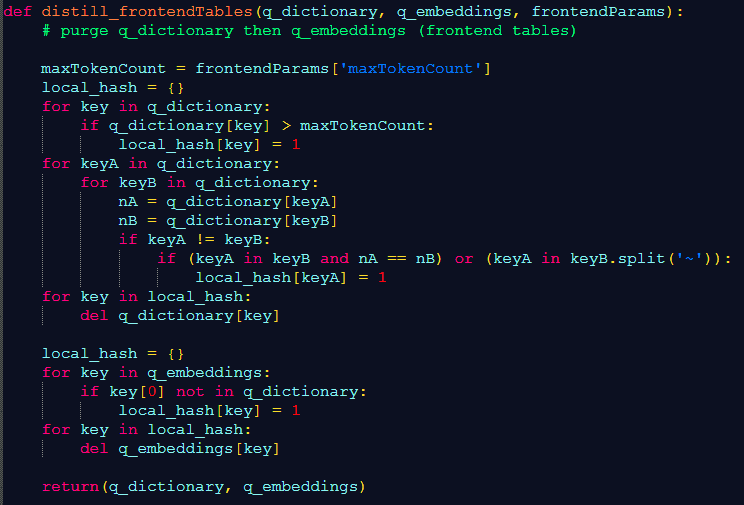
Analysis of the Developments in AI
The key points from the text hint at important trends and challenges in AI, including reproducibility, AI explainability, model distillation, variable-length embeddings, and real-time fine-tuning. Additionally, there is an apparent shift away from transformer-based models and independent AI training. Let’s discuss these aspects further and provide some insightful advice on the future implications in the AI landscape.
Reproducibility and AI Explainability
In the current AI landscape, reproducibility and explainability are becoming increasingly critical. With AI applications permeating all sectors, incorrect predictions can have severe consequences. Ensuring AI models are explainable makes it easier to identify and fix any bias, incorrect assumptions, or errors. Furthermore, reproducibility ensures that AI models consistently provide reliable results.
Model Distillation and Variable-Length Embeddings
Model distillation enables the transfer of knowledge from large, cumbersome models to smaller, faster ones. On the other hand, variable-length embeddings pave the way towards more efficient and flexible AI systems. With the increasing demand for efficient AI systems, these techniques will play a crucial role in future AI development.
Long-Term Implications
Increasing Importance of No-Transformer Models
With the shift away from transformer-based models hinted in the text, there seems to be a push towards simpler, faster models that can provide real-time feedback. This shift complements the growing trend of on-device AI, which requires models to be efficient and lightweight while maintaining high performance.
Shift Towards Real-time Fine-Tuning
Real-time fine-tuning refers to dynamically adjusting the AI model during inference, which is driven by the need for models to adapt to new data quickly. This technique can make AI more responsive, adaptable, and efficient, especially in rapidly changing environments.
Possible Future Developments
AI without Independent Training
The decline of independent AI training suggests a future where AI models learn and improve continuously, not through separate training phases. Continuous learning systems could adapt better to changing environments and respond more accurately to dynamic user demands.
Actionable Advice
- Focus on Explainability and Reproducibility: Developers should put more emphasis on ensuring AI models are explainable, to build trust with users, and reproducible, to maintain the reliability of results.
- Invest in Model Distillation and Variable-Length Embeddings: As AI continues to expand into real-world applications, investing in techniques for model distillation and exploring variable-length embeddings might help cope with the demand for more efficient systems.
- Explore alternatives to Transformer Models: Consider researching non-transformer-based models for efficient, high-performance systems, especially for edge computing applications.
- Prioritize Continuous Learning and Real-Time Fine Tuning: Given the dynamic nature of many real-world contexts, AI models that can respond and adapt in real-time will become increasingly important.